mirror of
https://github.com/VictoriaMetrics/VictoriaMetrics.git
synced 2025-01-07 00:22:16 +01:00
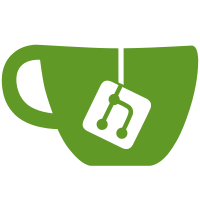
Previously empty series (e.g. series with all NaN samples) were passed to aggregate functions. Such series must be ingored by all the aggregate functions. So it is better from consistency PoV filtering out empty series before applying aggregate functions.
1158 lines
27 KiB
Go
1158 lines
27 KiB
Go
package promql
|
|
|
|
import (
|
|
"fmt"
|
|
"math"
|
|
"sort"
|
|
"strconv"
|
|
"strings"
|
|
|
|
"github.com/VictoriaMetrics/VictoriaMetrics/lib/logger"
|
|
"github.com/VictoriaMetrics/VictoriaMetrics/lib/storage"
|
|
"github.com/VictoriaMetrics/metrics"
|
|
"github.com/VictoriaMetrics/metricsql"
|
|
"github.com/cespare/xxhash/v2"
|
|
)
|
|
|
|
var aggrFuncs = map[string]aggrFunc{
|
|
"any": aggrFuncAny,
|
|
"avg": newAggrFunc(aggrFuncAvg),
|
|
"bottomk": newAggrFuncTopK(true),
|
|
"bottomk_avg": newAggrFuncRangeTopK(avgValue, true),
|
|
"bottomk_max": newAggrFuncRangeTopK(maxValue, true),
|
|
"bottomk_median": newAggrFuncRangeTopK(medianValue, true),
|
|
"bottomk_last": newAggrFuncRangeTopK(lastValue, true),
|
|
"bottomk_min": newAggrFuncRangeTopK(minValue, true),
|
|
"count": newAggrFunc(aggrFuncCount),
|
|
"count_values": aggrFuncCountValues,
|
|
"distinct": newAggrFunc(aggrFuncDistinct),
|
|
"geomean": newAggrFunc(aggrFuncGeomean),
|
|
"group": newAggrFunc(aggrFuncGroup),
|
|
"histogram": newAggrFunc(aggrFuncHistogram),
|
|
"limitk": aggrFuncLimitK,
|
|
"mad": newAggrFunc(aggrFuncMAD),
|
|
"max": newAggrFunc(aggrFuncMax),
|
|
"median": aggrFuncMedian,
|
|
"min": newAggrFunc(aggrFuncMin),
|
|
"mode": newAggrFunc(aggrFuncMode),
|
|
"outliers_mad": aggrFuncOutliersMAD,
|
|
"outliersk": aggrFuncOutliersK,
|
|
"quantile": aggrFuncQuantile,
|
|
"quantiles": aggrFuncQuantiles,
|
|
"stddev": newAggrFunc(aggrFuncStddev),
|
|
"stdvar": newAggrFunc(aggrFuncStdvar),
|
|
"sum": newAggrFunc(aggrFuncSum),
|
|
"sum2": newAggrFunc(aggrFuncSum2),
|
|
"topk": newAggrFuncTopK(false),
|
|
"topk_avg": newAggrFuncRangeTopK(avgValue, false),
|
|
"topk_max": newAggrFuncRangeTopK(maxValue, false),
|
|
"topk_median": newAggrFuncRangeTopK(medianValue, false),
|
|
"topk_last": newAggrFuncRangeTopK(lastValue, false),
|
|
"topk_min": newAggrFuncRangeTopK(minValue, false),
|
|
"zscore": aggrFuncZScore,
|
|
}
|
|
|
|
type aggrFunc func(afa *aggrFuncArg) ([]*timeseries, error)
|
|
|
|
type aggrFuncArg struct {
|
|
args [][]*timeseries
|
|
ae *metricsql.AggrFuncExpr
|
|
ec *EvalConfig
|
|
}
|
|
|
|
func getAggrFunc(s string) aggrFunc {
|
|
s = strings.ToLower(s)
|
|
return aggrFuncs[s]
|
|
}
|
|
|
|
func newAggrFunc(afe func(tss []*timeseries) []*timeseries) aggrFunc {
|
|
return func(afa *aggrFuncArg) ([]*timeseries, error) {
|
|
tss, err := getAggrTimeseries(afa.args)
|
|
if err != nil {
|
|
return nil, err
|
|
}
|
|
return aggrFuncExt(func(tss []*timeseries, modififer *metricsql.ModifierExpr) []*timeseries {
|
|
return afe(tss)
|
|
}, tss, &afa.ae.Modifier, afa.ae.Limit, false)
|
|
}
|
|
}
|
|
|
|
func getAggrTimeseries(args [][]*timeseries) ([]*timeseries, error) {
|
|
if len(args) == 0 {
|
|
return nil, fmt.Errorf("expecting at least one arg")
|
|
}
|
|
tss := args[0]
|
|
for _, arg := range args[1:] {
|
|
tss = append(tss, arg...)
|
|
}
|
|
return tss, nil
|
|
}
|
|
|
|
func removeGroupTags(metricName *storage.MetricName, modifier *metricsql.ModifierExpr) {
|
|
groupOp := strings.ToLower(modifier.Op)
|
|
switch groupOp {
|
|
case "", "by":
|
|
metricName.RemoveTagsOn(modifier.Args)
|
|
case "without":
|
|
metricName.RemoveTagsIgnoring(modifier.Args)
|
|
// Reset metric group as Prometheus does on `aggr(...) without (...)` call.
|
|
metricName.ResetMetricGroup()
|
|
default:
|
|
logger.Panicf("BUG: unknown group modifier: %q", groupOp)
|
|
}
|
|
}
|
|
|
|
func aggrFuncExt(afe func(tss []*timeseries, modifier *metricsql.ModifierExpr) []*timeseries, argOrig []*timeseries,
|
|
modifier *metricsql.ModifierExpr, maxSeries int, keepOriginal bool) ([]*timeseries, error) {
|
|
// Remove empty time series, e.g. series with all NaN samples,
|
|
// since such series are ignored by aggregate functions.
|
|
argOrig = removeEmptySeries(argOrig)
|
|
arg := copyTimeseriesMetricNames(argOrig, keepOriginal)
|
|
|
|
// Perform grouping.
|
|
m := make(map[string][]*timeseries)
|
|
bb := bbPool.Get()
|
|
for i, ts := range arg {
|
|
removeGroupTags(&ts.MetricName, modifier)
|
|
bb.B = marshalMetricNameSorted(bb.B[:0], &ts.MetricName)
|
|
if keepOriginal {
|
|
ts = argOrig[i]
|
|
}
|
|
tss := m[string(bb.B)]
|
|
if tss == nil && maxSeries > 0 && len(m) >= maxSeries {
|
|
// We already reached time series limit after grouping. Skip other time series.
|
|
continue
|
|
}
|
|
tss = append(tss, ts)
|
|
m[string(bb.B)] = tss
|
|
}
|
|
bbPool.Put(bb)
|
|
|
|
srcTssCount := 0
|
|
dstTssCount := 0
|
|
rvs := make([]*timeseries, 0, len(m))
|
|
for _, tss := range m {
|
|
rv := afe(tss, modifier)
|
|
rvs = append(rvs, rv...)
|
|
srcTssCount += len(tss)
|
|
dstTssCount += len(rv)
|
|
if dstTssCount > 2000 && dstTssCount > 16*srcTssCount {
|
|
// This looks like count_values explosion.
|
|
return nil, fmt.Errorf(`too many timeseries after aggragation; got %d; want less than %d`, dstTssCount, 16*srcTssCount)
|
|
}
|
|
}
|
|
return rvs, nil
|
|
}
|
|
|
|
func aggrFuncAny(afa *aggrFuncArg) ([]*timeseries, error) {
|
|
tss, err := getAggrTimeseries(afa.args)
|
|
if err != nil {
|
|
return nil, err
|
|
}
|
|
afe := func(tss []*timeseries, modifier *metricsql.ModifierExpr) []*timeseries {
|
|
return tss[:1]
|
|
}
|
|
limit := afa.ae.Limit
|
|
if limit > 1 {
|
|
// Only a single time series per group must be returned
|
|
limit = 1
|
|
}
|
|
return aggrFuncExt(afe, tss, &afa.ae.Modifier, limit, true)
|
|
}
|
|
|
|
func aggrFuncGroup(tss []*timeseries) []*timeseries {
|
|
// See https://github.com/prometheus/prometheus/commit/72425d4e3d14d209cc3f3f6e10e3240411303399
|
|
dst := tss[0]
|
|
for i := range dst.Values {
|
|
v := nan
|
|
for _, ts := range tss {
|
|
if math.IsNaN(ts.Values[i]) {
|
|
continue
|
|
}
|
|
v = 1
|
|
}
|
|
dst.Values[i] = v
|
|
}
|
|
return tss[:1]
|
|
}
|
|
|
|
func aggrFuncSum(tss []*timeseries) []*timeseries {
|
|
if len(tss) == 1 {
|
|
// Fast path - nothing to sum.
|
|
return tss
|
|
}
|
|
dst := tss[0]
|
|
for i := range dst.Values {
|
|
sum := float64(0)
|
|
count := 0
|
|
for _, ts := range tss {
|
|
v := ts.Values[i]
|
|
if math.IsNaN(v) {
|
|
continue
|
|
}
|
|
sum += v
|
|
count++
|
|
}
|
|
if count == 0 {
|
|
sum = nan
|
|
}
|
|
dst.Values[i] = sum
|
|
}
|
|
return tss[:1]
|
|
}
|
|
|
|
func aggrFuncSum2(tss []*timeseries) []*timeseries {
|
|
dst := tss[0]
|
|
for i := range dst.Values {
|
|
sum2 := float64(0)
|
|
count := 0
|
|
for _, ts := range tss {
|
|
v := ts.Values[i]
|
|
if math.IsNaN(v) {
|
|
continue
|
|
}
|
|
sum2 += v * v
|
|
count++
|
|
}
|
|
if count == 0 {
|
|
sum2 = nan
|
|
}
|
|
dst.Values[i] = sum2
|
|
}
|
|
return tss[:1]
|
|
}
|
|
|
|
func aggrFuncGeomean(tss []*timeseries) []*timeseries {
|
|
if len(tss) == 1 {
|
|
// Fast path - nothing to geomean.
|
|
return tss
|
|
}
|
|
dst := tss[0]
|
|
for i := range dst.Values {
|
|
p := 1.0
|
|
count := 0
|
|
for _, ts := range tss {
|
|
v := ts.Values[i]
|
|
if math.IsNaN(v) {
|
|
continue
|
|
}
|
|
p *= v
|
|
count++
|
|
}
|
|
if count == 0 {
|
|
p = nan
|
|
}
|
|
dst.Values[i] = math.Pow(p, 1/float64(count))
|
|
}
|
|
return tss[:1]
|
|
}
|
|
|
|
func aggrFuncHistogram(tss []*timeseries) []*timeseries {
|
|
var h metrics.Histogram
|
|
m := make(map[string]*timeseries)
|
|
for i := range tss[0].Values {
|
|
h.Reset()
|
|
for _, ts := range tss {
|
|
v := ts.Values[i]
|
|
h.Update(v)
|
|
}
|
|
h.VisitNonZeroBuckets(func(vmrange string, count uint64) {
|
|
ts := m[vmrange]
|
|
if ts == nil {
|
|
ts = ×eries{}
|
|
ts.CopyFromShallowTimestamps(tss[0])
|
|
ts.MetricName.RemoveTag("vmrange")
|
|
ts.MetricName.AddTag("vmrange", vmrange)
|
|
values := ts.Values
|
|
for k := range values {
|
|
values[k] = 0
|
|
}
|
|
m[vmrange] = ts
|
|
}
|
|
ts.Values[i] = float64(count)
|
|
})
|
|
}
|
|
rvs := make([]*timeseries, 0, len(m))
|
|
for _, ts := range m {
|
|
rvs = append(rvs, ts)
|
|
}
|
|
return vmrangeBucketsToLE(rvs)
|
|
}
|
|
|
|
func aggrFuncMin(tss []*timeseries) []*timeseries {
|
|
if len(tss) == 1 {
|
|
// Fast path - nothing to min.
|
|
return tss
|
|
}
|
|
dst := tss[0]
|
|
for i := range dst.Values {
|
|
min := dst.Values[i]
|
|
for _, ts := range tss {
|
|
if math.IsNaN(min) || ts.Values[i] < min {
|
|
min = ts.Values[i]
|
|
}
|
|
}
|
|
dst.Values[i] = min
|
|
}
|
|
return tss[:1]
|
|
}
|
|
|
|
func aggrFuncMax(tss []*timeseries) []*timeseries {
|
|
if len(tss) == 1 {
|
|
// Fast path - nothing to max.
|
|
return tss
|
|
}
|
|
dst := tss[0]
|
|
for i := range dst.Values {
|
|
max := dst.Values[i]
|
|
for _, ts := range tss {
|
|
if math.IsNaN(max) || ts.Values[i] > max {
|
|
max = ts.Values[i]
|
|
}
|
|
}
|
|
dst.Values[i] = max
|
|
}
|
|
return tss[:1]
|
|
}
|
|
|
|
func aggrFuncAvg(tss []*timeseries) []*timeseries {
|
|
if len(tss) == 1 {
|
|
// Fast path - nothing to avg.
|
|
return tss
|
|
}
|
|
dst := tss[0]
|
|
for i := range dst.Values {
|
|
// Do not use `Rapid calculation methods` at https://en.wikipedia.org/wiki/Standard_deviation,
|
|
// since it is slower and has no obvious benefits in increased precision.
|
|
var sum float64
|
|
count := 0
|
|
for _, ts := range tss {
|
|
v := ts.Values[i]
|
|
if math.IsNaN(v) {
|
|
continue
|
|
}
|
|
count++
|
|
sum += v
|
|
}
|
|
avg := nan
|
|
if count > 0 {
|
|
avg = sum / float64(count)
|
|
}
|
|
dst.Values[i] = avg
|
|
}
|
|
return tss[:1]
|
|
}
|
|
|
|
func aggrFuncStddev(tss []*timeseries) []*timeseries {
|
|
if len(tss) == 1 {
|
|
// Fast path - stddev over a single time series is zero
|
|
values := tss[0].Values
|
|
for i, v := range values {
|
|
if !math.IsNaN(v) {
|
|
values[i] = 0
|
|
}
|
|
}
|
|
return tss
|
|
}
|
|
rvs := aggrFuncStdvar(tss)
|
|
dst := rvs[0]
|
|
for i, v := range dst.Values {
|
|
dst.Values[i] = math.Sqrt(v)
|
|
}
|
|
return rvs
|
|
}
|
|
|
|
func aggrFuncStdvar(tss []*timeseries) []*timeseries {
|
|
if len(tss) == 1 {
|
|
// Fast path - stdvar over a single time series is zero
|
|
values := tss[0].Values
|
|
for i, v := range values {
|
|
if !math.IsNaN(v) {
|
|
values[i] = 0
|
|
}
|
|
}
|
|
return tss
|
|
}
|
|
dst := tss[0]
|
|
for i := range dst.Values {
|
|
// See `Rapid calculation methods` at https://en.wikipedia.org/wiki/Standard_deviation
|
|
var avg, count, q float64
|
|
for _, ts := range tss {
|
|
v := ts.Values[i]
|
|
if math.IsNaN(v) {
|
|
continue
|
|
}
|
|
count++
|
|
avgNew := avg + (v-avg)/count
|
|
q += (v - avg) * (v - avgNew)
|
|
avg = avgNew
|
|
}
|
|
if count == 0 {
|
|
q = nan
|
|
}
|
|
dst.Values[i] = q / count
|
|
}
|
|
return tss[:1]
|
|
}
|
|
|
|
func aggrFuncCount(tss []*timeseries) []*timeseries {
|
|
dst := tss[0]
|
|
for i := range dst.Values {
|
|
count := 0
|
|
for _, ts := range tss {
|
|
if math.IsNaN(ts.Values[i]) {
|
|
continue
|
|
}
|
|
count++
|
|
}
|
|
v := float64(count)
|
|
if count == 0 {
|
|
v = nan
|
|
}
|
|
dst.Values[i] = v
|
|
}
|
|
return tss[:1]
|
|
}
|
|
|
|
func aggrFuncDistinct(tss []*timeseries) []*timeseries {
|
|
dst := tss[0]
|
|
m := make(map[float64]struct{}, len(tss))
|
|
for i := range dst.Values {
|
|
for _, ts := range tss {
|
|
v := ts.Values[i]
|
|
if math.IsNaN(v) {
|
|
continue
|
|
}
|
|
m[v] = struct{}{}
|
|
}
|
|
n := float64(len(m))
|
|
if n == 0 {
|
|
n = nan
|
|
}
|
|
dst.Values[i] = n
|
|
for k := range m {
|
|
delete(m, k)
|
|
}
|
|
}
|
|
return tss[:1]
|
|
}
|
|
|
|
func aggrFuncMode(tss []*timeseries) []*timeseries {
|
|
dst := tss[0]
|
|
a := make([]float64, 0, len(tss))
|
|
for i := range dst.Values {
|
|
a := a[:0]
|
|
for _, ts := range tss {
|
|
v := ts.Values[i]
|
|
if !math.IsNaN(v) {
|
|
a = append(a, v)
|
|
}
|
|
}
|
|
dst.Values[i] = modeNoNaNs(nan, a)
|
|
}
|
|
return tss[:1]
|
|
}
|
|
|
|
func aggrFuncZScore(afa *aggrFuncArg) ([]*timeseries, error) {
|
|
tss, err := getAggrTimeseries(afa.args)
|
|
if err != nil {
|
|
return nil, err
|
|
}
|
|
afe := func(tss []*timeseries, modifier *metricsql.ModifierExpr) []*timeseries {
|
|
for i := range tss[0].Values {
|
|
// Calculate avg and stddev for tss points at position i.
|
|
// See `Rapid calculation methods` at https://en.wikipedia.org/wiki/Standard_deviation
|
|
var avg, count, q float64
|
|
for _, ts := range tss {
|
|
v := ts.Values[i]
|
|
if math.IsNaN(v) {
|
|
continue
|
|
}
|
|
count++
|
|
avgNew := avg + (v-avg)/count
|
|
q += (v - avg) * (v - avgNew)
|
|
avg = avgNew
|
|
}
|
|
if count == 0 {
|
|
// Cannot calculate z-score for NaN points.
|
|
continue
|
|
}
|
|
|
|
// Calculate z-score for tss points at position i.
|
|
// See https://en.wikipedia.org/wiki/Standard_score
|
|
stddev := math.Sqrt(q / count)
|
|
for _, ts := range tss {
|
|
v := ts.Values[i]
|
|
if math.IsNaN(v) {
|
|
continue
|
|
}
|
|
ts.Values[i] = (v - avg) / stddev
|
|
}
|
|
}
|
|
// Remove MetricGroup from all the tss.
|
|
for _, ts := range tss {
|
|
ts.MetricName.ResetMetricGroup()
|
|
}
|
|
return tss
|
|
}
|
|
return aggrFuncExt(afe, tss, &afa.ae.Modifier, afa.ae.Limit, true)
|
|
}
|
|
|
|
// modeNoNaNs returns mode for a.
|
|
//
|
|
// It is expected that a doesn't contain NaNs.
|
|
//
|
|
// The function modifies contents for a, so the caller must prepare it accordingly.
|
|
//
|
|
// See https://en.wikipedia.org/wiki/Mode_(statistics)
|
|
func modeNoNaNs(prevValue float64, a []float64) float64 {
|
|
if len(a) == 0 {
|
|
return prevValue
|
|
}
|
|
sort.Float64s(a)
|
|
j := -1
|
|
dMax := 0
|
|
mode := prevValue
|
|
for i, v := range a {
|
|
if prevValue == v {
|
|
continue
|
|
}
|
|
if d := i - j; d > dMax || math.IsNaN(mode) {
|
|
dMax = d
|
|
mode = prevValue
|
|
}
|
|
j = i
|
|
prevValue = v
|
|
}
|
|
if d := len(a) - j; d > dMax || math.IsNaN(mode) {
|
|
mode = prevValue
|
|
}
|
|
return mode
|
|
}
|
|
|
|
func aggrFuncCountValues(afa *aggrFuncArg) ([]*timeseries, error) {
|
|
args := afa.args
|
|
if err := expectTransformArgsNum(args, 2); err != nil {
|
|
return nil, err
|
|
}
|
|
dstLabel, err := getString(args[0], 0)
|
|
if err != nil {
|
|
return nil, err
|
|
}
|
|
|
|
// Remove dstLabel from grouping like Prometheus does.
|
|
modifier := &afa.ae.Modifier
|
|
switch strings.ToLower(modifier.Op) {
|
|
case "without":
|
|
modifier.Args = append(modifier.Args, dstLabel)
|
|
case "by":
|
|
dstArgs := modifier.Args[:0]
|
|
for _, arg := range modifier.Args {
|
|
if arg == dstLabel {
|
|
continue
|
|
}
|
|
dstArgs = append(dstArgs, arg)
|
|
}
|
|
modifier.Args = dstArgs
|
|
default:
|
|
// Do nothing
|
|
}
|
|
|
|
afe := func(tss []*timeseries, modififer *metricsql.ModifierExpr) []*timeseries {
|
|
m := make(map[float64]bool)
|
|
for _, ts := range tss {
|
|
for _, v := range ts.Values {
|
|
if math.IsNaN(v) {
|
|
continue
|
|
}
|
|
m[v] = true
|
|
}
|
|
}
|
|
values := make([]float64, 0, len(m))
|
|
for v := range m {
|
|
values = append(values, v)
|
|
}
|
|
sort.Float64s(values)
|
|
|
|
var rvs []*timeseries
|
|
for _, v := range values {
|
|
var dst timeseries
|
|
dst.CopyFromShallowTimestamps(tss[0])
|
|
dst.MetricName.RemoveTag(dstLabel)
|
|
dst.MetricName.AddTag(dstLabel, strconv.FormatFloat(v, 'f', -1, 64))
|
|
for i := range dst.Values {
|
|
count := 0
|
|
for _, ts := range tss {
|
|
if ts.Values[i] == v {
|
|
count++
|
|
}
|
|
}
|
|
n := float64(count)
|
|
if n == 0 {
|
|
n = nan
|
|
}
|
|
dst.Values[i] = n
|
|
}
|
|
rvs = append(rvs, &dst)
|
|
}
|
|
return rvs
|
|
}
|
|
return aggrFuncExt(afe, args[1], &afa.ae.Modifier, afa.ae.Limit, false)
|
|
}
|
|
|
|
func newAggrFuncTopK(isReverse bool) aggrFunc {
|
|
return func(afa *aggrFuncArg) ([]*timeseries, error) {
|
|
args := afa.args
|
|
if err := expectTransformArgsNum(args, 2); err != nil {
|
|
return nil, err
|
|
}
|
|
ks, err := getScalar(args[0], 0)
|
|
if err != nil {
|
|
return nil, err
|
|
}
|
|
afe := func(tss []*timeseries, modififer *metricsql.ModifierExpr) []*timeseries {
|
|
for n := range tss[0].Values {
|
|
sort.Slice(tss, func(i, j int) bool {
|
|
a := tss[i].Values[n]
|
|
b := tss[j].Values[n]
|
|
if isReverse {
|
|
a, b = b, a
|
|
}
|
|
return lessWithNaNs(a, b)
|
|
})
|
|
fillNaNsAtIdx(n, ks[n], tss)
|
|
}
|
|
tss = removeEmptySeries(tss)
|
|
reverseSeries(tss)
|
|
return tss
|
|
}
|
|
return aggrFuncExt(afe, args[1], &afa.ae.Modifier, afa.ae.Limit, true)
|
|
}
|
|
}
|
|
|
|
func newAggrFuncRangeTopK(f func(values []float64) float64, isReverse bool) aggrFunc {
|
|
return func(afa *aggrFuncArg) ([]*timeseries, error) {
|
|
args := afa.args
|
|
if len(args) < 2 {
|
|
return nil, fmt.Errorf(`unexpected number of args; got %d; want at least %d`, len(args), 2)
|
|
}
|
|
if len(args) > 3 {
|
|
return nil, fmt.Errorf(`unexpected number of args; got %d; want no more than %d`, len(args), 3)
|
|
}
|
|
ks, err := getScalar(args[0], 0)
|
|
if err != nil {
|
|
return nil, err
|
|
}
|
|
remainingSumTagName := ""
|
|
if len(args) == 3 {
|
|
remainingSumTagName, err = getString(args[2], 2)
|
|
if err != nil {
|
|
return nil, err
|
|
}
|
|
}
|
|
afe := func(tss []*timeseries, modifier *metricsql.ModifierExpr) []*timeseries {
|
|
return getRangeTopKTimeseries(tss, modifier, ks, remainingSumTagName, f, isReverse)
|
|
}
|
|
return aggrFuncExt(afe, args[1], &afa.ae.Modifier, afa.ae.Limit, true)
|
|
}
|
|
}
|
|
|
|
func getRangeTopKTimeseries(tss []*timeseries, modifier *metricsql.ModifierExpr, ks []float64, remainingSumTagName string,
|
|
f func(values []float64) float64, isReverse bool) []*timeseries {
|
|
type tsWithValue struct {
|
|
ts *timeseries
|
|
value float64
|
|
}
|
|
maxs := make([]tsWithValue, len(tss))
|
|
for i, ts := range tss {
|
|
value := f(ts.Values)
|
|
maxs[i] = tsWithValue{
|
|
ts: ts,
|
|
value: value,
|
|
}
|
|
}
|
|
sort.Slice(maxs, func(i, j int) bool {
|
|
a := maxs[i].value
|
|
b := maxs[j].value
|
|
if isReverse {
|
|
a, b = b, a
|
|
}
|
|
return lessWithNaNs(a, b)
|
|
})
|
|
for i := range maxs {
|
|
tss[i] = maxs[i].ts
|
|
}
|
|
remainingSumTS := getRemainingSumTimeseries(tss, modifier, ks, remainingSumTagName)
|
|
for i, k := range ks {
|
|
fillNaNsAtIdx(i, k, tss)
|
|
}
|
|
if remainingSumTS != nil {
|
|
tss = append(tss, remainingSumTS)
|
|
}
|
|
tss = removeEmptySeries(tss)
|
|
reverseSeries(tss)
|
|
return tss
|
|
}
|
|
|
|
func reverseSeries(tss []*timeseries) {
|
|
j := len(tss)
|
|
for i := 0; i < len(tss)/2; i++ {
|
|
j--
|
|
tss[i], tss[j] = tss[j], tss[i]
|
|
}
|
|
}
|
|
|
|
func getRemainingSumTimeseries(tss []*timeseries, modifier *metricsql.ModifierExpr, ks []float64, remainingSumTagName string) *timeseries {
|
|
if len(remainingSumTagName) == 0 || len(tss) == 0 {
|
|
return nil
|
|
}
|
|
var dst timeseries
|
|
dst.CopyFromShallowTimestamps(tss[0])
|
|
removeGroupTags(&dst.MetricName, modifier)
|
|
tagValue := remainingSumTagName
|
|
n := strings.IndexByte(remainingSumTagName, '=')
|
|
if n >= 0 {
|
|
tagValue = remainingSumTagName[n+1:]
|
|
remainingSumTagName = remainingSumTagName[:n]
|
|
}
|
|
dst.MetricName.RemoveTag(remainingSumTagName)
|
|
dst.MetricName.AddTag(remainingSumTagName, tagValue)
|
|
for i, k := range ks {
|
|
kn := getIntK(k, len(tss))
|
|
var sum float64
|
|
count := 0
|
|
for _, ts := range tss[:len(tss)-kn] {
|
|
v := ts.Values[i]
|
|
if math.IsNaN(v) {
|
|
continue
|
|
}
|
|
sum += v
|
|
count++
|
|
}
|
|
if count == 0 {
|
|
sum = nan
|
|
}
|
|
dst.Values[i] = sum
|
|
}
|
|
return &dst
|
|
}
|
|
|
|
func fillNaNsAtIdx(idx int, k float64, tss []*timeseries) {
|
|
kn := getIntK(k, len(tss))
|
|
for _, ts := range tss[:len(tss)-kn] {
|
|
ts.Values[idx] = nan
|
|
}
|
|
}
|
|
|
|
func getIntK(k float64, kMax int) int {
|
|
if math.IsNaN(k) {
|
|
return 0
|
|
}
|
|
kn := int(k)
|
|
if kn < 0 {
|
|
return 0
|
|
}
|
|
if kn > kMax {
|
|
return kMax
|
|
}
|
|
return kn
|
|
}
|
|
|
|
func minValue(values []float64) float64 {
|
|
min := nan
|
|
for len(values) > 0 && math.IsNaN(min) {
|
|
min = values[0]
|
|
values = values[1:]
|
|
}
|
|
for _, v := range values {
|
|
if !math.IsNaN(v) && v < min {
|
|
min = v
|
|
}
|
|
}
|
|
return min
|
|
}
|
|
|
|
func maxValue(values []float64) float64 {
|
|
max := nan
|
|
for len(values) > 0 && math.IsNaN(max) {
|
|
max = values[0]
|
|
values = values[1:]
|
|
}
|
|
for _, v := range values {
|
|
if !math.IsNaN(v) && v > max {
|
|
max = v
|
|
}
|
|
}
|
|
return max
|
|
}
|
|
|
|
func avgValue(values []float64) float64 {
|
|
sum := float64(0)
|
|
count := 0
|
|
for _, v := range values {
|
|
if math.IsNaN(v) {
|
|
continue
|
|
}
|
|
count++
|
|
sum += v
|
|
}
|
|
if count == 0 {
|
|
return nan
|
|
}
|
|
return sum / float64(count)
|
|
}
|
|
|
|
func medianValue(values []float64) float64 {
|
|
return quantile(0.5, values)
|
|
}
|
|
|
|
func lastValue(values []float64) float64 {
|
|
values = skipTrailingNaNs(values)
|
|
if len(values) == 0 {
|
|
return nan
|
|
}
|
|
return values[len(values)-1]
|
|
}
|
|
|
|
// quantiles calculates the given phis from originValues without modifying originValues, appends them to qs and returns the result.
|
|
func quantiles(qs, phis []float64, originValues []float64) []float64 {
|
|
a := getFloat64s()
|
|
a.A = prepareForQuantileFloat64(a.A[:0], originValues)
|
|
qs = quantilesSorted(qs, phis, a.A)
|
|
putFloat64s(a)
|
|
return qs
|
|
}
|
|
|
|
// quantile calculates the given phi from originValues without modifying originValues
|
|
func quantile(phi float64, originValues []float64) float64 {
|
|
a := getFloat64s()
|
|
a.A = prepareForQuantileFloat64(a.A[:0], originValues)
|
|
q := quantileSorted(phi, a.A)
|
|
putFloat64s(a)
|
|
return q
|
|
}
|
|
|
|
// prepareForQuantileFloat64 copies items from src to dst but removes NaNs and sorts the dst
|
|
func prepareForQuantileFloat64(dst, src []float64) []float64 {
|
|
for _, v := range src {
|
|
if math.IsNaN(v) {
|
|
continue
|
|
}
|
|
dst = append(dst, v)
|
|
}
|
|
sort.Float64s(dst)
|
|
return dst
|
|
}
|
|
|
|
// quantilesSorted calculates the given phis over a sorted list of values, appends them to qs and returns the result.
|
|
//
|
|
// It is expected that values won't contain NaN items.
|
|
// The implementation mimics Prometheus implementation for compatibility's sake.
|
|
func quantilesSorted(qs, phis []float64, values []float64) []float64 {
|
|
for _, phi := range phis {
|
|
q := quantileSorted(phi, values)
|
|
qs = append(qs, q)
|
|
}
|
|
return qs
|
|
}
|
|
|
|
// quantileSorted calculates the given quantile over a sorted list of values.
|
|
//
|
|
// It is expected that values won't contain NaN items.
|
|
// The implementation mimics Prometheus implementation for compatibility's sake.
|
|
func quantileSorted(phi float64, values []float64) float64 {
|
|
if len(values) == 0 || math.IsNaN(phi) {
|
|
return nan
|
|
}
|
|
if phi < 0 {
|
|
return math.Inf(-1)
|
|
}
|
|
if phi > 1 {
|
|
return math.Inf(+1)
|
|
}
|
|
n := float64(len(values))
|
|
rank := phi * (n - 1)
|
|
|
|
lowerIndex := math.Max(0, math.Floor(rank))
|
|
upperIndex := math.Min(n-1, lowerIndex+1)
|
|
|
|
weight := rank - math.Floor(rank)
|
|
return values[int(lowerIndex)]*(1-weight) + values[int(upperIndex)]*weight
|
|
}
|
|
|
|
func aggrFuncMAD(tss []*timeseries) []*timeseries {
|
|
// Calculate medians for each point across tss.
|
|
medians := getPerPointMedians(tss)
|
|
// Calculate MAD values multipled by tolerance for each point across tss.
|
|
// See https://en.wikipedia.org/wiki/Median_absolute_deviation
|
|
mads := getPerPointMADs(tss, medians)
|
|
tss[0].Values = append(tss[0].Values[:0], mads...)
|
|
return tss[:1]
|
|
}
|
|
|
|
func aggrFuncOutliersMAD(afa *aggrFuncArg) ([]*timeseries, error) {
|
|
args := afa.args
|
|
if err := expectTransformArgsNum(args, 2); err != nil {
|
|
return nil, err
|
|
}
|
|
tolerances, err := getScalar(args[0], 0)
|
|
if err != nil {
|
|
return nil, err
|
|
}
|
|
afe := func(tss []*timeseries, modifier *metricsql.ModifierExpr) []*timeseries {
|
|
// Calculate medians for each point across tss.
|
|
medians := getPerPointMedians(tss)
|
|
// Calculate MAD values multipled by tolerance for each point across tss.
|
|
// See https://en.wikipedia.org/wiki/Median_absolute_deviation
|
|
mads := getPerPointMADs(tss, medians)
|
|
for n := range mads {
|
|
mads[n] *= tolerances[n]
|
|
}
|
|
// Leave only time series with at least a single peak above the MAD multiplied by tolerance.
|
|
tssDst := tss[:0]
|
|
for _, ts := range tss {
|
|
values := ts.Values
|
|
for n, v := range values {
|
|
ad := math.Abs(v - medians[n])
|
|
mad := mads[n]
|
|
if ad > mad {
|
|
tssDst = append(tssDst, ts)
|
|
break
|
|
}
|
|
}
|
|
}
|
|
return tssDst
|
|
}
|
|
return aggrFuncExt(afe, args[1], &afa.ae.Modifier, afa.ae.Limit, true)
|
|
}
|
|
|
|
func aggrFuncOutliersK(afa *aggrFuncArg) ([]*timeseries, error) {
|
|
args := afa.args
|
|
if err := expectTransformArgsNum(args, 2); err != nil {
|
|
return nil, err
|
|
}
|
|
ks, err := getScalar(args[0], 0)
|
|
if err != nil {
|
|
return nil, err
|
|
}
|
|
afe := func(tss []*timeseries, modifier *metricsql.ModifierExpr) []*timeseries {
|
|
// Calculate medians for each point across tss.
|
|
medians := getPerPointMedians(tss)
|
|
// Return topK time series with the highest variance from median.
|
|
f := func(values []float64) float64 {
|
|
sum2 := float64(0)
|
|
for n, v := range values {
|
|
d := v - medians[n]
|
|
sum2 += d * d
|
|
}
|
|
return sum2
|
|
}
|
|
return getRangeTopKTimeseries(tss, &afa.ae.Modifier, ks, "", f, false)
|
|
}
|
|
return aggrFuncExt(afe, args[1], &afa.ae.Modifier, afa.ae.Limit, true)
|
|
}
|
|
|
|
func getPerPointMedians(tss []*timeseries) []float64 {
|
|
if len(tss) == 0 {
|
|
logger.Panicf("BUG: expecting non-empty tss")
|
|
}
|
|
medians := make([]float64, len(tss[0].Values))
|
|
a := getFloat64s()
|
|
values := a.A
|
|
for n := range medians {
|
|
values = values[:0]
|
|
for j := range tss {
|
|
v := tss[j].Values[n]
|
|
if !math.IsNaN(v) {
|
|
values = append(values, v)
|
|
}
|
|
}
|
|
medians[n] = quantile(0.5, values)
|
|
}
|
|
a.A = values
|
|
putFloat64s(a)
|
|
return medians
|
|
}
|
|
|
|
func getPerPointMADs(tss []*timeseries, medians []float64) []float64 {
|
|
mads := make([]float64, len(medians))
|
|
a := getFloat64s()
|
|
values := a.A
|
|
for n, median := range medians {
|
|
values = values[:0]
|
|
for j := range tss {
|
|
v := tss[j].Values[n]
|
|
if !math.IsNaN(v) {
|
|
ad := math.Abs(v - median)
|
|
values = append(values, ad)
|
|
}
|
|
}
|
|
mads[n] = quantile(0.5, values)
|
|
}
|
|
a.A = values
|
|
putFloat64s(a)
|
|
return mads
|
|
}
|
|
|
|
func aggrFuncLimitK(afa *aggrFuncArg) ([]*timeseries, error) {
|
|
args := afa.args
|
|
if err := expectTransformArgsNum(args, 2); err != nil {
|
|
return nil, err
|
|
}
|
|
limits, err := getScalar(args[0], 0)
|
|
if err != nil {
|
|
return nil, fmt.Errorf("cannot obtain limit arg: %w", err)
|
|
}
|
|
limit := 0
|
|
if len(limits) > 0 {
|
|
limit = int(limits[0])
|
|
}
|
|
if limit < 0 {
|
|
limit = 0
|
|
}
|
|
afe := func(tss []*timeseries, modifier *metricsql.ModifierExpr) []*timeseries {
|
|
// Sort series by metricName hash in order to get consistent set of output series
|
|
// across multiple calls to limitk() function.
|
|
// Sort series by hash in order to guarantee uniform selection across series.
|
|
type hashSeries struct {
|
|
h uint64
|
|
ts *timeseries
|
|
}
|
|
hss := make([]hashSeries, len(tss))
|
|
d := xxhash.New()
|
|
for i, ts := range tss {
|
|
h := getHash(d, &ts.MetricName)
|
|
hss[i] = hashSeries{
|
|
h: h,
|
|
ts: ts,
|
|
}
|
|
}
|
|
sort.Slice(hss, func(i, j int) bool {
|
|
return hss[i].h < hss[j].h
|
|
})
|
|
for i, hs := range hss {
|
|
tss[i] = hs.ts
|
|
}
|
|
if limit < len(tss) {
|
|
tss = tss[:limit]
|
|
}
|
|
return tss
|
|
}
|
|
return aggrFuncExt(afe, args[1], &afa.ae.Modifier, afa.ae.Limit, true)
|
|
}
|
|
|
|
func getHash(d *xxhash.Digest, mn *storage.MetricName) uint64 {
|
|
d.Reset()
|
|
_, _ = d.Write(mn.MetricGroup)
|
|
for _, tag := range mn.Tags {
|
|
_, _ = d.Write(tag.Key)
|
|
_, _ = d.Write(tag.Value)
|
|
}
|
|
return d.Sum64()
|
|
|
|
}
|
|
|
|
func aggrFuncQuantiles(afa *aggrFuncArg) ([]*timeseries, error) {
|
|
args := afa.args
|
|
if len(args) < 3 {
|
|
return nil, fmt.Errorf("unexpected number of args: %d; expecting at least 3 args", len(args))
|
|
}
|
|
dstLabel, err := getString(args[0], 0)
|
|
if err != nil {
|
|
return nil, fmt.Errorf("cannot obtain dstLabel: %w", err)
|
|
}
|
|
phiArgs := args[1 : len(args)-1]
|
|
phis := make([]float64, len(phiArgs))
|
|
for i, phiArg := range phiArgs {
|
|
phisLocal, err := getScalar(phiArg, i+1)
|
|
if err != nil {
|
|
return nil, err
|
|
}
|
|
if len(phis) == 0 {
|
|
logger.Panicf("BUG: expecting at least a single sample")
|
|
}
|
|
phis[i] = phisLocal[0]
|
|
}
|
|
argOrig := args[len(args)-1]
|
|
afe := func(tss []*timeseries, modifier *metricsql.ModifierExpr) []*timeseries {
|
|
tssDst := make([]*timeseries, len(phiArgs))
|
|
for j := range tssDst {
|
|
ts := ×eries{}
|
|
ts.CopyFromShallowTimestamps(tss[0])
|
|
ts.MetricName.RemoveTag(dstLabel)
|
|
ts.MetricName.AddTag(dstLabel, fmt.Sprintf("%g", phis[j]))
|
|
tssDst[j] = ts
|
|
}
|
|
|
|
b := getFloat64s()
|
|
qs := b.A
|
|
a := getFloat64s()
|
|
values := a.A
|
|
for n := range tss[0].Values {
|
|
values = values[:0]
|
|
for j := range tss {
|
|
values = append(values, tss[j].Values[n])
|
|
}
|
|
qs = quantiles(qs[:0], phis, values)
|
|
for j := range tssDst {
|
|
tssDst[j].Values[n] = qs[j]
|
|
}
|
|
}
|
|
a.A = values
|
|
putFloat64s(a)
|
|
b.A = qs
|
|
putFloat64s(b)
|
|
return tssDst
|
|
}
|
|
return aggrFuncExt(afe, argOrig, &afa.ae.Modifier, afa.ae.Limit, false)
|
|
}
|
|
|
|
func aggrFuncQuantile(afa *aggrFuncArg) ([]*timeseries, error) {
|
|
args := afa.args
|
|
if err := expectTransformArgsNum(args, 2); err != nil {
|
|
return nil, err
|
|
}
|
|
phis, err := getScalar(args[0], 0)
|
|
if err != nil {
|
|
return nil, err
|
|
}
|
|
afe := newAggrQuantileFunc(phis)
|
|
return aggrFuncExt(afe, args[1], &afa.ae.Modifier, afa.ae.Limit, false)
|
|
}
|
|
|
|
func aggrFuncMedian(afa *aggrFuncArg) ([]*timeseries, error) {
|
|
tss, err := getAggrTimeseries(afa.args)
|
|
if err != nil {
|
|
return nil, err
|
|
}
|
|
phis := evalNumber(afa.ec, 0.5)[0].Values
|
|
afe := newAggrQuantileFunc(phis)
|
|
return aggrFuncExt(afe, tss, &afa.ae.Modifier, afa.ae.Limit, false)
|
|
}
|
|
|
|
func newAggrQuantileFunc(phis []float64) func(tss []*timeseries, modifier *metricsql.ModifierExpr) []*timeseries {
|
|
return func(tss []*timeseries, modifier *metricsql.ModifierExpr) []*timeseries {
|
|
dst := tss[0]
|
|
a := getFloat64s()
|
|
values := a.A
|
|
for n := range dst.Values {
|
|
values = values[:0]
|
|
for j := range tss {
|
|
values = append(values, tss[j].Values[n])
|
|
}
|
|
dst.Values[n] = quantile(phis[n], values)
|
|
}
|
|
a.A = values
|
|
putFloat64s(a)
|
|
tss[0] = dst
|
|
return tss[:1]
|
|
}
|
|
}
|
|
|
|
func lessWithNaNs(a, b float64) bool {
|
|
if math.IsNaN(a) {
|
|
return !math.IsNaN(b)
|
|
}
|
|
return a < b
|
|
}
|