mirror of
https://github.com/VictoriaMetrics/VictoriaMetrics.git
synced 2025-01-12 05:28:13 +01:00
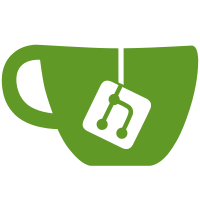
Previously too short lookbehind window d for rate(m[d]) could be automatically extended if it didn't cover at least two raw samples. This was needed in order to guarantee non-empty results from rate(m[d]) on short time ranges. Now the lookbehind window isn't extended if it is set explicitly, since it is expected that the user knows what he is doing. The lookbehind window continues to be extended when needed if it isn't set explicitly. For example, in the case of rate(m). Updates https://github.com/VictoriaMetrics/VictoriaMetrics/issues/3483
2177 lines
60 KiB
Go
2177 lines
60 KiB
Go
package promql
|
|
|
|
import (
|
|
"flag"
|
|
"fmt"
|
|
"math"
|
|
"strings"
|
|
"sync"
|
|
|
|
"github.com/VictoriaMetrics/VictoriaMetrics/lib/decimal"
|
|
"github.com/VictoriaMetrics/VictoriaMetrics/lib/logger"
|
|
"github.com/VictoriaMetrics/VictoriaMetrics/lib/storage"
|
|
"github.com/VictoriaMetrics/metrics"
|
|
"github.com/VictoriaMetrics/metricsql"
|
|
)
|
|
|
|
var minStalenessInterval = flag.Duration("search.minStalenessInterval", 0, "The minimum interval for staleness calculations. "+
|
|
"This flag could be useful for removing gaps on graphs generated from time series with irregular intervals between samples. "+
|
|
"See also '-search.maxStalenessInterval'")
|
|
|
|
var rollupFuncs = map[string]newRollupFunc{
|
|
"absent_over_time": newRollupFuncOneArg(rollupAbsent),
|
|
"aggr_over_time": newRollupFuncTwoArgs(rollupFake),
|
|
"ascent_over_time": newRollupFuncOneArg(rollupAscentOverTime),
|
|
"avg_over_time": newRollupFuncOneArg(rollupAvg),
|
|
"changes": newRollupFuncOneArg(rollupChanges),
|
|
"changes_prometheus": newRollupFuncOneArg(rollupChangesPrometheus),
|
|
"count_eq_over_time": newRollupCountEQ,
|
|
"count_gt_over_time": newRollupCountGT,
|
|
"count_le_over_time": newRollupCountLE,
|
|
"count_ne_over_time": newRollupCountNE,
|
|
"count_over_time": newRollupFuncOneArg(rollupCount),
|
|
"decreases_over_time": newRollupFuncOneArg(rollupDecreases),
|
|
"default_rollup": newRollupFuncOneArg(rollupDefault), // default rollup func
|
|
"delta": newRollupFuncOneArg(rollupDelta),
|
|
"delta_prometheus": newRollupFuncOneArg(rollupDeltaPrometheus),
|
|
"deriv": newRollupFuncOneArg(rollupDerivSlow),
|
|
"deriv_fast": newRollupFuncOneArg(rollupDerivFast),
|
|
"descent_over_time": newRollupFuncOneArg(rollupDescentOverTime),
|
|
"distinct_over_time": newRollupFuncOneArg(rollupDistinct),
|
|
"duration_over_time": newRollupDurationOverTime,
|
|
"first_over_time": newRollupFuncOneArg(rollupFirst),
|
|
"geomean_over_time": newRollupFuncOneArg(rollupGeomean),
|
|
"histogram_over_time": newRollupFuncOneArg(rollupHistogram),
|
|
"hoeffding_bound_lower": newRollupHoeffdingBoundLower,
|
|
"hoeffding_bound_upper": newRollupHoeffdingBoundUpper,
|
|
"holt_winters": newRollupHoltWinters,
|
|
"idelta": newRollupFuncOneArg(rollupIdelta),
|
|
"ideriv": newRollupFuncOneArg(rollupIderiv),
|
|
"increase": newRollupFuncOneArg(rollupDelta), // + rollupFuncsRemoveCounterResets
|
|
"increase_prometheus": newRollupFuncOneArg(rollupDeltaPrometheus), // + rollupFuncsRemoveCounterResets
|
|
"increase_pure": newRollupFuncOneArg(rollupIncreasePure), // + rollupFuncsRemoveCounterResets
|
|
"increases_over_time": newRollupFuncOneArg(rollupIncreases),
|
|
"integrate": newRollupFuncOneArg(rollupIntegrate),
|
|
"irate": newRollupFuncOneArg(rollupIderiv), // + rollupFuncsRemoveCounterResets
|
|
"lag": newRollupFuncOneArg(rollupLag),
|
|
"last_over_time": newRollupFuncOneArg(rollupLast),
|
|
"lifetime": newRollupFuncOneArg(rollupLifetime),
|
|
"max_over_time": newRollupFuncOneArg(rollupMax),
|
|
"min_over_time": newRollupFuncOneArg(rollupMin),
|
|
"mode_over_time": newRollupFuncOneArg(rollupModeOverTime),
|
|
"predict_linear": newRollupPredictLinear,
|
|
"present_over_time": newRollupFuncOneArg(rollupPresent),
|
|
"quantile_over_time": newRollupQuantile,
|
|
"quantiles_over_time": newRollupQuantiles,
|
|
"range_over_time": newRollupFuncOneArg(rollupRange),
|
|
"rate": newRollupFuncOneArg(rollupDerivFast), // + rollupFuncsRemoveCounterResets
|
|
"rate_over_sum": newRollupFuncOneArg(rollupRateOverSum),
|
|
"resets": newRollupFuncOneArg(rollupResets),
|
|
"rollup": newRollupFuncOneArg(rollupFake),
|
|
"rollup_candlestick": newRollupFuncOneArg(rollupFake),
|
|
"rollup_delta": newRollupFuncOneArg(rollupFake),
|
|
"rollup_deriv": newRollupFuncOneArg(rollupFake),
|
|
"rollup_increase": newRollupFuncOneArg(rollupFake), // + rollupFuncsRemoveCounterResets
|
|
"rollup_rate": newRollupFuncOneArg(rollupFake), // + rollupFuncsRemoveCounterResets
|
|
"rollup_scrape_interval": newRollupFuncOneArg(rollupFake),
|
|
"scrape_interval": newRollupFuncOneArg(rollupScrapeInterval),
|
|
"share_gt_over_time": newRollupShareGT,
|
|
"share_le_over_time": newRollupShareLE,
|
|
"stale_samples_over_time": newRollupFuncOneArg(rollupStaleSamples),
|
|
"stddev_over_time": newRollupFuncOneArg(rollupStddev),
|
|
"stdvar_over_time": newRollupFuncOneArg(rollupStdvar),
|
|
"sum_over_time": newRollupFuncOneArg(rollupSum),
|
|
"sum2_over_time": newRollupFuncOneArg(rollupSum2),
|
|
"tfirst_over_time": newRollupFuncOneArg(rollupTfirst),
|
|
// `timestamp` function must return timestamp for the last datapoint on the current window
|
|
// in order to properly handle offset and timestamps unaligned to the current step.
|
|
// See https://github.com/VictoriaMetrics/VictoriaMetrics/issues/415 for details.
|
|
"timestamp": newRollupFuncOneArg(rollupTlast),
|
|
"timestamp_with_name": newRollupFuncOneArg(rollupTlast), // + rollupFuncsKeepMetricName
|
|
"tlast_change_over_time": newRollupFuncOneArg(rollupTlastChange),
|
|
"tlast_over_time": newRollupFuncOneArg(rollupTlast),
|
|
"tmax_over_time": newRollupFuncOneArg(rollupTmax),
|
|
"tmin_over_time": newRollupFuncOneArg(rollupTmin),
|
|
"zscore_over_time": newRollupFuncOneArg(rollupZScoreOverTime),
|
|
}
|
|
|
|
// rollupAggrFuncs are functions that can be passed to `aggr_over_time()`
|
|
var rollupAggrFuncs = map[string]rollupFunc{
|
|
"absent_over_time": rollupAbsent,
|
|
"ascent_over_time": rollupAscentOverTime,
|
|
"avg_over_time": rollupAvg,
|
|
"changes": rollupChanges,
|
|
"count_over_time": rollupCount,
|
|
"decreases_over_time": rollupDecreases,
|
|
"default_rollup": rollupDefault,
|
|
"delta": rollupDelta,
|
|
"deriv": rollupDerivSlow,
|
|
"deriv_fast": rollupDerivFast,
|
|
"descent_over_time": rollupDescentOverTime,
|
|
"distinct_over_time": rollupDistinct,
|
|
"first_over_time": rollupFirst,
|
|
"geomean_over_time": rollupGeomean,
|
|
"idelta": rollupIdelta,
|
|
"ideriv": rollupIderiv,
|
|
"increase": rollupDelta,
|
|
"increase_pure": rollupIncreasePure,
|
|
"increases_over_time": rollupIncreases,
|
|
"integrate": rollupIntegrate,
|
|
"irate": rollupIderiv,
|
|
"lag": rollupLag,
|
|
"last_over_time": rollupLast,
|
|
"lifetime": rollupLifetime,
|
|
"max_over_time": rollupMax,
|
|
"min_over_time": rollupMin,
|
|
"mode_over_time": rollupModeOverTime,
|
|
"present_over_time": rollupPresent,
|
|
"range_over_time": rollupRange,
|
|
"rate": rollupDerivFast,
|
|
"rate_over_sum": rollupRateOverSum,
|
|
"resets": rollupResets,
|
|
"scrape_interval": rollupScrapeInterval,
|
|
"stale_samples_over_time": rollupStaleSamples,
|
|
"stddev_over_time": rollupStddev,
|
|
"stdvar_over_time": rollupStdvar,
|
|
"sum_over_time": rollupSum,
|
|
"sum2_over_time": rollupSum2,
|
|
"tfirst_over_time": rollupTfirst,
|
|
"timestamp": rollupTlast,
|
|
"timestamp_with_name": rollupTlast,
|
|
"tlast_change_over_time": rollupTlastChange,
|
|
"tlast_over_time": rollupTlast,
|
|
"tmax_over_time": rollupTmax,
|
|
"tmin_over_time": rollupTmin,
|
|
"zscore_over_time": rollupZScoreOverTime,
|
|
}
|
|
|
|
// VictoriaMetrics can extends lookbehind window for these functions
|
|
// in order to make sure it contains enough points for returning non-empty results.
|
|
//
|
|
// This is needed for returning the expected non-empty graphs when zooming in the graph in Grafana,
|
|
// which is built with `func_name(metric)` query.
|
|
var rollupFuncsCanAdjustWindow = map[string]bool{
|
|
"default_rollup": true,
|
|
"deriv": true,
|
|
"deriv_fast": true,
|
|
"ideriv": true,
|
|
"irate": true,
|
|
"rate": true,
|
|
"rate_over_sum": true,
|
|
"rollup": true,
|
|
"rollup_candlestick": true,
|
|
"rollup_deriv": true,
|
|
"rollup_rate": true,
|
|
"rollup_scrape_interval": true,
|
|
"scrape_interval": true,
|
|
"timestamp": true,
|
|
}
|
|
|
|
// rollupFuncsRemoveCounterResets contains functions, which need to call removeCounterResets
|
|
// over input samples before calling the corresponding rollup functions.
|
|
var rollupFuncsRemoveCounterResets = map[string]bool{
|
|
"increase": true,
|
|
"increase_prometheus": true,
|
|
"increase_pure": true,
|
|
"irate": true,
|
|
"rate": true,
|
|
"rollup_increase": true,
|
|
"rollup_rate": true,
|
|
}
|
|
|
|
// rollupFuncsSamplesScannedPerCall contains functions, which scan lower number of samples
|
|
// than is passed to the rollup func.
|
|
//
|
|
// It is expected that the remaining rollupFuncs scan all the samples passed to them.
|
|
var rollupFuncsSamplesScannedPerCall = map[string]int{
|
|
"absent_over_time": 1,
|
|
"count_over_time": 1,
|
|
"default_rollup": 1,
|
|
"delta": 2,
|
|
"delta_prometheus": 2,
|
|
"deriv_fast": 2,
|
|
"first_over_time": 1,
|
|
"idelta": 2,
|
|
"ideriv": 2,
|
|
"increase": 2,
|
|
"increase_prometheus": 2,
|
|
"increase_pure": 2,
|
|
"irate": 2,
|
|
"lag": 1,
|
|
"last_over_time": 1,
|
|
"lifetime": 2,
|
|
"present_over_time": 1,
|
|
"rate": 2,
|
|
"scrape_interval": 2,
|
|
"tfirst_over_time": 1,
|
|
"timestamp": 1,
|
|
"timestamp_with_name": 1,
|
|
"tlast_over_time": 1,
|
|
}
|
|
|
|
// These functions don't change physical meaning of input time series,
|
|
// so they don't drop metric name
|
|
var rollupFuncsKeepMetricName = map[string]bool{
|
|
"avg_over_time": true,
|
|
"default_rollup": true,
|
|
"first_over_time": true,
|
|
"geomean_over_time": true,
|
|
"hoeffding_bound_lower": true,
|
|
"hoeffding_bound_upper": true,
|
|
"holt_winters": true,
|
|
"last_over_time": true,
|
|
"max_over_time": true,
|
|
"min_over_time": true,
|
|
"mode_over_time": true,
|
|
"predict_linear": true,
|
|
"quantile_over_time": true,
|
|
"quantiles_over_time": true,
|
|
"rollup": true,
|
|
"rollup_candlestick": true,
|
|
"timestamp_with_name": true,
|
|
}
|
|
|
|
func getRollupAggrFuncNames(expr metricsql.Expr) ([]string, error) {
|
|
afe, ok := expr.(*metricsql.AggrFuncExpr)
|
|
if ok {
|
|
// This is for incremental aggregate function case:
|
|
//
|
|
// sum(aggr_over_time(...))
|
|
//
|
|
// See aggr_incremental.go for details.
|
|
expr = afe.Args[0]
|
|
}
|
|
fe, ok := expr.(*metricsql.FuncExpr)
|
|
if !ok {
|
|
logger.Panicf("BUG: unexpected expression; want metricsql.FuncExpr; got %T; value: %s", expr, expr.AppendString(nil))
|
|
}
|
|
if fe.Name != "aggr_over_time" {
|
|
logger.Panicf("BUG: unexpected function name: %q; want `aggr_over_time`", fe.Name)
|
|
}
|
|
if len(fe.Args) != 2 {
|
|
return nil, fmt.Errorf("unexpected number of args to aggr_over_time(); got %d; want %d", len(fe.Args), 2)
|
|
}
|
|
arg := fe.Args[0]
|
|
var aggrFuncNames []string
|
|
if se, ok := arg.(*metricsql.StringExpr); ok {
|
|
aggrFuncNames = append(aggrFuncNames, se.S)
|
|
} else {
|
|
fe, ok := arg.(*metricsql.FuncExpr)
|
|
if !ok || fe.Name != "" {
|
|
return nil, fmt.Errorf("%s cannot be passed to aggr_over_time(); expecting quoted aggregate function name or a list of quoted aggregate function names",
|
|
arg.AppendString(nil))
|
|
}
|
|
for _, e := range fe.Args {
|
|
se, ok := e.(*metricsql.StringExpr)
|
|
if !ok {
|
|
return nil, fmt.Errorf("%s cannot be passed here; expecting quoted aggregate function name", e.AppendString(nil))
|
|
}
|
|
aggrFuncNames = append(aggrFuncNames, se.S)
|
|
}
|
|
}
|
|
if len(aggrFuncNames) == 0 {
|
|
return nil, fmt.Errorf("aggr_over_time() must contain at least a single aggregate function name")
|
|
}
|
|
for _, s := range aggrFuncNames {
|
|
if rollupAggrFuncs[s] == nil {
|
|
return nil, fmt.Errorf("%q cannot be used in `aggr_over_time` function; expecting quoted aggregate function name", s)
|
|
}
|
|
}
|
|
return aggrFuncNames, nil
|
|
}
|
|
|
|
func getRollupConfigs(funcName string, rf rollupFunc, expr metricsql.Expr, start, end, step int64, maxPointsPerSeries int,
|
|
window, lookbackDelta int64, sharedTimestamps []int64) (
|
|
func(values []float64, timestamps []int64), []*rollupConfig, error) {
|
|
preFunc := func(values []float64, timestamps []int64) {}
|
|
funcName = strings.ToLower(funcName)
|
|
if rollupFuncsRemoveCounterResets[funcName] {
|
|
preFunc = func(values []float64, timestamps []int64) {
|
|
removeCounterResets(values)
|
|
}
|
|
}
|
|
samplesScannedPerCall := rollupFuncsSamplesScannedPerCall[funcName]
|
|
newRollupConfig := func(rf rollupFunc, tagValue string) *rollupConfig {
|
|
return &rollupConfig{
|
|
TagValue: tagValue,
|
|
Func: rf,
|
|
Start: start,
|
|
End: end,
|
|
Step: step,
|
|
Window: window,
|
|
|
|
MaxPointsPerSeries: maxPointsPerSeries,
|
|
|
|
MayAdjustWindow: rollupFuncsCanAdjustWindow[funcName],
|
|
LookbackDelta: lookbackDelta,
|
|
Timestamps: sharedTimestamps,
|
|
isDefaultRollup: funcName == "default_rollup",
|
|
samplesScannedPerCall: samplesScannedPerCall,
|
|
}
|
|
}
|
|
appendRollupConfigs := func(dst []*rollupConfig) []*rollupConfig {
|
|
dst = append(dst, newRollupConfig(rollupMin, "min"))
|
|
dst = append(dst, newRollupConfig(rollupMax, "max"))
|
|
dst = append(dst, newRollupConfig(rollupAvg, "avg"))
|
|
return dst
|
|
}
|
|
var rcs []*rollupConfig
|
|
switch funcName {
|
|
case "rollup":
|
|
rcs = appendRollupConfigs(rcs)
|
|
case "rollup_rate", "rollup_deriv":
|
|
preFuncPrev := preFunc
|
|
preFunc = func(values []float64, timestamps []int64) {
|
|
preFuncPrev(values, timestamps)
|
|
derivValues(values, timestamps)
|
|
}
|
|
rcs = appendRollupConfigs(rcs)
|
|
case "rollup_increase", "rollup_delta":
|
|
preFuncPrev := preFunc
|
|
preFunc = func(values []float64, timestamps []int64) {
|
|
preFuncPrev(values, timestamps)
|
|
deltaValues(values)
|
|
}
|
|
rcs = appendRollupConfigs(rcs)
|
|
case "rollup_candlestick":
|
|
rcs = append(rcs, newRollupConfig(rollupOpen, "open"))
|
|
rcs = append(rcs, newRollupConfig(rollupClose, "close"))
|
|
rcs = append(rcs, newRollupConfig(rollupLow, "low"))
|
|
rcs = append(rcs, newRollupConfig(rollupHigh, "high"))
|
|
case "rollup_scrape_interval":
|
|
preFuncPrev := preFunc
|
|
preFunc = func(values []float64, timestamps []int64) {
|
|
preFuncPrev(values, timestamps)
|
|
// Calculate intervals in seconds between samples.
|
|
tsSecsPrev := nan
|
|
for i, ts := range timestamps {
|
|
tsSecs := float64(ts) / 1000
|
|
values[i] = tsSecs - tsSecsPrev
|
|
tsSecsPrev = tsSecs
|
|
}
|
|
if len(values) > 1 {
|
|
// Overwrite the first NaN interval with the second interval,
|
|
// So min, max and avg rollups could be calculated properly, since they don't expect to receive NaNs.
|
|
values[0] = values[1]
|
|
}
|
|
}
|
|
rcs = appendRollupConfigs(rcs)
|
|
case "aggr_over_time":
|
|
aggrFuncNames, err := getRollupAggrFuncNames(expr)
|
|
if err != nil {
|
|
return nil, nil, fmt.Errorf("invalid args to %s: %w", expr.AppendString(nil), err)
|
|
}
|
|
for _, aggrFuncName := range aggrFuncNames {
|
|
if rollupFuncsRemoveCounterResets[aggrFuncName] {
|
|
// There is no need to save the previous preFunc, since it is either empty or the same.
|
|
preFunc = func(values []float64, timestamps []int64) {
|
|
removeCounterResets(values)
|
|
}
|
|
}
|
|
rf := rollupAggrFuncs[aggrFuncName]
|
|
rcs = append(rcs, newRollupConfig(rf, aggrFuncName))
|
|
}
|
|
default:
|
|
rcs = append(rcs, newRollupConfig(rf, ""))
|
|
}
|
|
return preFunc, rcs, nil
|
|
}
|
|
|
|
func getRollupFunc(funcName string) newRollupFunc {
|
|
funcName = strings.ToLower(funcName)
|
|
return rollupFuncs[funcName]
|
|
}
|
|
|
|
type rollupFuncArg struct {
|
|
// The value preceeding values if it fits staleness interval.
|
|
prevValue float64
|
|
|
|
// The timestamp for prevValue.
|
|
prevTimestamp int64
|
|
|
|
// Values that fit window ending at currTimestamp.
|
|
values []float64
|
|
|
|
// Timestamps for values.
|
|
timestamps []int64
|
|
|
|
// Real value preceeding values without restrictions on staleness interval.
|
|
realPrevValue float64
|
|
|
|
// Real value which goes after values.
|
|
realNextValue float64
|
|
|
|
// Current timestamp for rollup evaluation.
|
|
currTimestamp int64
|
|
|
|
// Index for the currently evaluated point relative to time range for query evaluation.
|
|
idx int
|
|
|
|
// Time window for rollup calculations.
|
|
window int64
|
|
|
|
tsm *timeseriesMap
|
|
}
|
|
|
|
func (rfa *rollupFuncArg) reset() {
|
|
rfa.prevValue = 0
|
|
rfa.prevTimestamp = 0
|
|
rfa.values = nil
|
|
rfa.timestamps = nil
|
|
rfa.currTimestamp = 0
|
|
rfa.idx = 0
|
|
rfa.window = 0
|
|
rfa.tsm = nil
|
|
}
|
|
|
|
// rollupFunc must return rollup value for the given rfa.
|
|
//
|
|
// prevValue may be nan, values and timestamps may be empty.
|
|
type rollupFunc func(rfa *rollupFuncArg) float64
|
|
|
|
type rollupConfig struct {
|
|
// This tag value must be added to "rollup" tag if non-empty.
|
|
TagValue string
|
|
|
|
Func rollupFunc
|
|
Start int64
|
|
End int64
|
|
Step int64
|
|
Window int64
|
|
|
|
// The maximum number of points, which can be generated per each series.
|
|
MaxPointsPerSeries int
|
|
|
|
// Whether window may be adjusted to 2 x interval between data points.
|
|
// This is needed for functions which have dt in the denominator
|
|
// such as rate, deriv, etc.
|
|
// Without the adjustment their value would jump in unexpected directions
|
|
// when using window smaller than 2 x scrape_interval.
|
|
MayAdjustWindow bool
|
|
|
|
Timestamps []int64
|
|
|
|
// LoookbackDelta is the analog to `-query.lookback-delta` from Prometheus world.
|
|
LookbackDelta int64
|
|
|
|
// Whether default_rollup is used.
|
|
isDefaultRollup bool
|
|
|
|
// The estimated number of samples scanned per Func call.
|
|
//
|
|
// If zero, then it is considered that Func scans all the samples passed to it.
|
|
samplesScannedPerCall int
|
|
}
|
|
|
|
func (rc *rollupConfig) getTimestamps() []int64 {
|
|
return getTimestamps(rc.Start, rc.End, rc.Step, rc.MaxPointsPerSeries)
|
|
}
|
|
|
|
func (rc *rollupConfig) String() string {
|
|
start := storage.TimestampToHumanReadableFormat(rc.Start)
|
|
end := storage.TimestampToHumanReadableFormat(rc.End)
|
|
return fmt.Sprintf("timeRange=[%s..%s], step=%d, window=%d, points=%d", start, end, rc.Step, rc.Window, len(rc.Timestamps))
|
|
}
|
|
|
|
var (
|
|
nan = math.NaN()
|
|
inf = math.Inf(1)
|
|
)
|
|
|
|
// The maximum interval without previous rows.
|
|
const maxSilenceInterval = 5 * 60 * 1000
|
|
|
|
type timeseriesMap struct {
|
|
origin *timeseries
|
|
h metrics.Histogram
|
|
m map[string]*timeseries
|
|
}
|
|
|
|
func newTimeseriesMap(funcName string, keepMetricNames bool, sharedTimestamps []int64, mnSrc *storage.MetricName) *timeseriesMap {
|
|
funcName = strings.ToLower(funcName)
|
|
switch funcName {
|
|
case "histogram_over_time", "quantiles_over_time":
|
|
default:
|
|
return nil
|
|
}
|
|
|
|
values := make([]float64, len(sharedTimestamps))
|
|
for i := range values {
|
|
values[i] = nan
|
|
}
|
|
var origin timeseries
|
|
origin.MetricName.CopyFrom(mnSrc)
|
|
if !keepMetricNames && !rollupFuncsKeepMetricName[funcName] {
|
|
origin.MetricName.ResetMetricGroup()
|
|
}
|
|
origin.Timestamps = sharedTimestamps
|
|
origin.Values = values
|
|
return ×eriesMap{
|
|
origin: &origin,
|
|
m: make(map[string]*timeseries),
|
|
}
|
|
}
|
|
|
|
func (tsm *timeseriesMap) AppendTimeseriesTo(dst []*timeseries) []*timeseries {
|
|
for _, ts := range tsm.m {
|
|
dst = append(dst, ts)
|
|
}
|
|
return dst
|
|
}
|
|
|
|
func (tsm *timeseriesMap) GetOrCreateTimeseries(labelName, labelValue string) *timeseries {
|
|
ts := tsm.m[labelValue]
|
|
if ts != nil {
|
|
return ts
|
|
}
|
|
ts = ×eries{}
|
|
ts.CopyFromShallowTimestamps(tsm.origin)
|
|
ts.MetricName.RemoveTag(labelName)
|
|
ts.MetricName.AddTag(labelName, labelValue)
|
|
tsm.m[labelValue] = ts
|
|
return ts
|
|
}
|
|
|
|
// Do calculates rollups for the given timestamps and values, appends
|
|
// them to dstValues and returns results.
|
|
//
|
|
// rc.Timestamps are used as timestamps for dstValues.
|
|
//
|
|
// timestamps must cover time range [rc.Start - rc.Window - maxSilenceInterval ... rc.End].
|
|
//
|
|
// Do cannot be called from concurrent goroutines.
|
|
func (rc *rollupConfig) Do(dstValues []float64, values []float64, timestamps []int64) ([]float64, uint64) {
|
|
return rc.doInternal(dstValues, nil, values, timestamps)
|
|
}
|
|
|
|
// DoTimeseriesMap calculates rollups for the given timestamps and values and puts them to tsm.
|
|
func (rc *rollupConfig) DoTimeseriesMap(tsm *timeseriesMap, values []float64, timestamps []int64) uint64 {
|
|
ts := getTimeseries()
|
|
var samplesScanned uint64
|
|
ts.Values, samplesScanned = rc.doInternal(ts.Values[:0], tsm, values, timestamps)
|
|
putTimeseries(ts)
|
|
return samplesScanned
|
|
}
|
|
|
|
func (rc *rollupConfig) doInternal(dstValues []float64, tsm *timeseriesMap, values []float64, timestamps []int64) ([]float64, uint64) {
|
|
// Sanity checks.
|
|
if rc.Step <= 0 {
|
|
logger.Panicf("BUG: Step must be bigger than 0; got %d", rc.Step)
|
|
}
|
|
if rc.Start > rc.End {
|
|
logger.Panicf("BUG: Start cannot exceed End; got %d vs %d", rc.Start, rc.End)
|
|
}
|
|
if rc.Window < 0 {
|
|
logger.Panicf("BUG: Window must be non-negative; got %d", rc.Window)
|
|
}
|
|
if err := ValidateMaxPointsPerSeries(rc.Start, rc.End, rc.Step, rc.MaxPointsPerSeries); err != nil {
|
|
logger.Panicf("BUG: %s; this must be validated before the call to rollupConfig.Do", err)
|
|
}
|
|
|
|
// Extend dstValues in order to remove mallocs below.
|
|
dstValues = decimal.ExtendFloat64sCapacity(dstValues, len(rc.Timestamps))
|
|
|
|
scrapeInterval := getScrapeInterval(timestamps, rc.Step)
|
|
maxPrevInterval := getMaxPrevInterval(scrapeInterval)
|
|
if rc.LookbackDelta > 0 && maxPrevInterval > rc.LookbackDelta {
|
|
maxPrevInterval = rc.LookbackDelta
|
|
}
|
|
if *minStalenessInterval > 0 {
|
|
if msi := minStalenessInterval.Milliseconds(); msi > 0 && maxPrevInterval < msi {
|
|
maxPrevInterval = msi
|
|
}
|
|
}
|
|
window := rc.Window
|
|
if window <= 0 {
|
|
window = rc.Step
|
|
if rc.MayAdjustWindow && window < maxPrevInterval {
|
|
// Adjust lookbehind window only if it isn't set explicilty, e.g. rate(foo).
|
|
// In the case of missing lookbehind window it should be adjusted in order to return non-empty graph
|
|
// when the window doesn't cover at least two raw samples (this is what most users expect).
|
|
//
|
|
// If the user explicitly sets the lookbehind window to some fixed value, e.g. rate(foo[1s]),
|
|
// then it is expected he knows what he is doing. Do not adjust the lookbehind window then.
|
|
//
|
|
// See https://github.com/VictoriaMetrics/VictoriaMetrics/issues/3483
|
|
window = maxPrevInterval
|
|
}
|
|
if rc.isDefaultRollup && rc.LookbackDelta > 0 && window > rc.LookbackDelta {
|
|
// Implicit window exceeds -search.maxStalenessInterval, so limit it to -search.maxStalenessInterval
|
|
// according to https://github.com/VictoriaMetrics/VictoriaMetrics/issues/784
|
|
window = rc.LookbackDelta
|
|
}
|
|
}
|
|
rfa := getRollupFuncArg()
|
|
rfa.idx = 0
|
|
rfa.window = window
|
|
rfa.tsm = tsm
|
|
|
|
i := 0
|
|
j := 0
|
|
ni := 0
|
|
nj := 0
|
|
f := rc.Func
|
|
samplesScanned := uint64(len(values))
|
|
samplesScannedPerCall := uint64(rc.samplesScannedPerCall)
|
|
for _, tEnd := range rc.Timestamps {
|
|
tStart := tEnd - window
|
|
ni = seekFirstTimestampIdxAfter(timestamps[i:], tStart, ni)
|
|
i += ni
|
|
if j < i {
|
|
j = i
|
|
}
|
|
nj = seekFirstTimestampIdxAfter(timestamps[j:], tEnd, nj)
|
|
j += nj
|
|
|
|
rfa.prevValue = nan
|
|
rfa.prevTimestamp = tStart - maxPrevInterval
|
|
if i < len(timestamps) && i > 0 && timestamps[i-1] > rfa.prevTimestamp {
|
|
rfa.prevValue = values[i-1]
|
|
rfa.prevTimestamp = timestamps[i-1]
|
|
}
|
|
rfa.values = values[i:j]
|
|
rfa.timestamps = timestamps[i:j]
|
|
if i > 0 {
|
|
rfa.realPrevValue = values[i-1]
|
|
} else {
|
|
rfa.realPrevValue = nan
|
|
}
|
|
if j < len(values) {
|
|
rfa.realNextValue = values[j]
|
|
} else {
|
|
rfa.realNextValue = nan
|
|
}
|
|
rfa.currTimestamp = tEnd
|
|
value := f(rfa)
|
|
rfa.idx++
|
|
if samplesScannedPerCall > 0 {
|
|
samplesScanned += samplesScannedPerCall
|
|
} else {
|
|
samplesScanned += uint64(len(rfa.values))
|
|
}
|
|
dstValues = append(dstValues, value)
|
|
}
|
|
putRollupFuncArg(rfa)
|
|
|
|
return dstValues, samplesScanned
|
|
}
|
|
|
|
func seekFirstTimestampIdxAfter(timestamps []int64, seekTimestamp int64, nHint int) int {
|
|
if len(timestamps) == 0 || timestamps[0] > seekTimestamp {
|
|
return 0
|
|
}
|
|
startIdx := nHint - 2
|
|
if startIdx < 0 {
|
|
startIdx = 0
|
|
}
|
|
if startIdx >= len(timestamps) {
|
|
startIdx = len(timestamps) - 1
|
|
}
|
|
endIdx := nHint + 2
|
|
if endIdx > len(timestamps) {
|
|
endIdx = len(timestamps)
|
|
}
|
|
if startIdx > 0 && timestamps[startIdx] <= seekTimestamp {
|
|
timestamps = timestamps[startIdx:]
|
|
endIdx -= startIdx
|
|
} else {
|
|
startIdx = 0
|
|
}
|
|
if endIdx < len(timestamps) && timestamps[endIdx] > seekTimestamp {
|
|
timestamps = timestamps[:endIdx]
|
|
}
|
|
if len(timestamps) < 16 {
|
|
// Fast path: the number of timestamps to search is small, so scan them all.
|
|
for i, timestamp := range timestamps {
|
|
if timestamp > seekTimestamp {
|
|
return startIdx + i
|
|
}
|
|
}
|
|
return startIdx + len(timestamps)
|
|
}
|
|
// Slow path: too big len(timestamps), so use binary search.
|
|
i := binarySearchInt64(timestamps, seekTimestamp+1)
|
|
return startIdx + int(i)
|
|
}
|
|
|
|
func binarySearchInt64(a []int64, v int64) uint {
|
|
// Copy-pasted sort.Search from https://golang.org/src/sort/search.go?s=2246:2286#L49
|
|
i, j := uint(0), uint(len(a))
|
|
for i < j {
|
|
h := (i + j) >> 1
|
|
if h < uint(len(a)) && a[h] < v {
|
|
i = h + 1
|
|
} else {
|
|
j = h
|
|
}
|
|
}
|
|
return i
|
|
}
|
|
|
|
func getScrapeInterval(timestamps []int64, defaultInterval int64) int64 {
|
|
if len(timestamps) < 2 {
|
|
// can't calculate scrape interval with less than 2 timestamps
|
|
// return defaultInterval
|
|
return defaultInterval
|
|
}
|
|
|
|
// Estimate scrape interval as 0.6 quantile for the first 20 intervals.
|
|
tsPrev := timestamps[0]
|
|
timestamps = timestamps[1:]
|
|
if len(timestamps) > 20 {
|
|
timestamps = timestamps[:20]
|
|
}
|
|
a := getFloat64s()
|
|
intervals := a.A[:0]
|
|
for _, ts := range timestamps {
|
|
intervals = append(intervals, float64(ts-tsPrev))
|
|
tsPrev = ts
|
|
}
|
|
scrapeInterval := int64(quantile(0.6, intervals))
|
|
a.A = intervals
|
|
putFloat64s(a)
|
|
if scrapeInterval <= 0 {
|
|
return defaultInterval
|
|
}
|
|
return scrapeInterval
|
|
}
|
|
|
|
func getMaxPrevInterval(scrapeInterval int64) int64 {
|
|
// Increase scrapeInterval more for smaller scrape intervals in order to hide possible gaps
|
|
// when high jitter is present.
|
|
// See https://github.com/VictoriaMetrics/VictoriaMetrics/issues/139 .
|
|
if scrapeInterval <= 2*1000 {
|
|
return scrapeInterval + 4*scrapeInterval
|
|
}
|
|
if scrapeInterval <= 4*1000 {
|
|
return scrapeInterval + 2*scrapeInterval
|
|
}
|
|
if scrapeInterval <= 8*1000 {
|
|
return scrapeInterval + scrapeInterval
|
|
}
|
|
if scrapeInterval <= 16*1000 {
|
|
return scrapeInterval + scrapeInterval/2
|
|
}
|
|
if scrapeInterval <= 32*1000 {
|
|
return scrapeInterval + scrapeInterval/4
|
|
}
|
|
return scrapeInterval + scrapeInterval/8
|
|
}
|
|
|
|
func removeCounterResets(values []float64) {
|
|
// There is no need in handling NaNs here, since they are impossible
|
|
// on values from vmstorage.
|
|
if len(values) == 0 {
|
|
return
|
|
}
|
|
var correction float64
|
|
prevValue := values[0]
|
|
for i, v := range values {
|
|
d := v - prevValue
|
|
if d < 0 {
|
|
if (-d * 8) < prevValue {
|
|
// This is likely a partial counter reset.
|
|
// See https://github.com/VictoriaMetrics/VictoriaMetrics/issues/2787
|
|
correction += prevValue - v
|
|
} else {
|
|
correction += prevValue
|
|
}
|
|
}
|
|
prevValue = v
|
|
values[i] = v + correction
|
|
}
|
|
}
|
|
|
|
func deltaValues(values []float64) {
|
|
// There is no need in handling NaNs here, since they are impossible
|
|
// on values from vmstorage.
|
|
if len(values) == 0 {
|
|
return
|
|
}
|
|
prevDelta := float64(0)
|
|
prevValue := values[0]
|
|
for i, v := range values[1:] {
|
|
prevDelta = v - prevValue
|
|
values[i] = prevDelta
|
|
prevValue = v
|
|
}
|
|
values[len(values)-1] = prevDelta
|
|
}
|
|
|
|
func derivValues(values []float64, timestamps []int64) {
|
|
// There is no need in handling NaNs here, since they are impossible
|
|
// on values from vmstorage.
|
|
if len(values) == 0 {
|
|
return
|
|
}
|
|
prevDeriv := float64(0)
|
|
prevValue := values[0]
|
|
prevTs := timestamps[0]
|
|
for i, v := range values[1:] {
|
|
ts := timestamps[i+1]
|
|
if ts == prevTs {
|
|
// Use the previous value for duplicate timestamps.
|
|
values[i] = prevDeriv
|
|
continue
|
|
}
|
|
dt := float64(ts-prevTs) / 1e3
|
|
prevDeriv = (v - prevValue) / dt
|
|
values[i] = prevDeriv
|
|
prevValue = v
|
|
prevTs = ts
|
|
}
|
|
values[len(values)-1] = prevDeriv
|
|
}
|
|
|
|
type newRollupFunc func(args []interface{}) (rollupFunc, error)
|
|
|
|
func newRollupFuncOneArg(rf rollupFunc) newRollupFunc {
|
|
return func(args []interface{}) (rollupFunc, error) {
|
|
if err := expectRollupArgsNum(args, 1); err != nil {
|
|
return nil, err
|
|
}
|
|
return rf, nil
|
|
}
|
|
}
|
|
|
|
func newRollupFuncTwoArgs(rf rollupFunc) newRollupFunc {
|
|
return func(args []interface{}) (rollupFunc, error) {
|
|
if err := expectRollupArgsNum(args, 2); err != nil {
|
|
return nil, err
|
|
}
|
|
return rf, nil
|
|
}
|
|
}
|
|
|
|
func newRollupHoltWinters(args []interface{}) (rollupFunc, error) {
|
|
if err := expectRollupArgsNum(args, 3); err != nil {
|
|
return nil, err
|
|
}
|
|
sfs, err := getScalar(args[1], 1)
|
|
if err != nil {
|
|
return nil, err
|
|
}
|
|
tfs, err := getScalar(args[2], 2)
|
|
if err != nil {
|
|
return nil, err
|
|
}
|
|
rf := func(rfa *rollupFuncArg) float64 {
|
|
// There is no need in handling NaNs here, since they must be cleaned up
|
|
// before calling rollup funcs.
|
|
values := rfa.values
|
|
if len(values) == 0 {
|
|
return rfa.prevValue
|
|
}
|
|
sf := sfs[rfa.idx]
|
|
if sf <= 0 || sf >= 1 {
|
|
return nan
|
|
}
|
|
tf := tfs[rfa.idx]
|
|
if tf <= 0 || tf >= 1 {
|
|
return nan
|
|
}
|
|
|
|
// See https://en.wikipedia.org/wiki/Exponential_smoothing#Double_exponential_smoothing .
|
|
// TODO: determine whether this shit really works.
|
|
s0 := rfa.prevValue
|
|
if math.IsNaN(s0) {
|
|
s0 = values[0]
|
|
values = values[1:]
|
|
if len(values) == 0 {
|
|
return s0
|
|
}
|
|
}
|
|
b0 := values[0] - s0
|
|
for _, v := range values {
|
|
s1 := sf*v + (1-sf)*(s0+b0)
|
|
b1 := tf*(s1-s0) + (1-tf)*b0
|
|
s0 = s1
|
|
b0 = b1
|
|
}
|
|
return s0
|
|
}
|
|
return rf, nil
|
|
}
|
|
|
|
func newRollupPredictLinear(args []interface{}) (rollupFunc, error) {
|
|
if err := expectRollupArgsNum(args, 2); err != nil {
|
|
return nil, err
|
|
}
|
|
secs, err := getScalar(args[1], 1)
|
|
if err != nil {
|
|
return nil, err
|
|
}
|
|
rf := func(rfa *rollupFuncArg) float64 {
|
|
v, k := linearRegression(rfa.values, rfa.timestamps, rfa.currTimestamp)
|
|
if math.IsNaN(v) {
|
|
return nan
|
|
}
|
|
sec := secs[rfa.idx]
|
|
return v + k*sec
|
|
}
|
|
return rf, nil
|
|
}
|
|
|
|
func linearRegression(values []float64, timestamps []int64, interceptTime int64) (float64, float64) {
|
|
if len(values) == 0 {
|
|
return nan, nan
|
|
}
|
|
if areConstValues(values) {
|
|
return values[0], 0
|
|
}
|
|
|
|
// See https://en.wikipedia.org/wiki/Simple_linear_regression#Numerical_example
|
|
vSum := float64(0)
|
|
tSum := float64(0)
|
|
tvSum := float64(0)
|
|
ttSum := float64(0)
|
|
n := 0
|
|
for i, v := range values {
|
|
if math.IsNaN(v) {
|
|
continue
|
|
}
|
|
dt := float64(timestamps[i]-interceptTime) / 1e3
|
|
vSum += v
|
|
tSum += dt
|
|
tvSum += dt * v
|
|
ttSum += dt * dt
|
|
n++
|
|
}
|
|
if n == 0 {
|
|
return nan, nan
|
|
}
|
|
k := float64(0)
|
|
tDiff := ttSum - tSum*tSum/float64(n)
|
|
if math.Abs(tDiff) >= 1e-6 {
|
|
// Prevent from incorrect division for too small tDiff values.
|
|
k = (tvSum - tSum*vSum/float64(n)) / tDiff
|
|
}
|
|
v := vSum/float64(n) - k*tSum/float64(n)
|
|
return v, k
|
|
}
|
|
|
|
func areConstValues(values []float64) bool {
|
|
if len(values) <= 1 {
|
|
return true
|
|
}
|
|
vPrev := values[0]
|
|
for _, v := range values[1:] {
|
|
if v != vPrev {
|
|
return false
|
|
}
|
|
vPrev = v
|
|
}
|
|
return true
|
|
}
|
|
|
|
func newRollupDurationOverTime(args []interface{}) (rollupFunc, error) {
|
|
if err := expectRollupArgsNum(args, 2); err != nil {
|
|
return nil, err
|
|
}
|
|
dMaxs, err := getScalar(args[1], 1)
|
|
if err != nil {
|
|
return nil, err
|
|
}
|
|
rf := func(rfa *rollupFuncArg) float64 {
|
|
// There is no need in handling NaNs here, since they must be cleaned up
|
|
// before calling rollup funcs.
|
|
timestamps := rfa.timestamps
|
|
if len(timestamps) == 0 {
|
|
return nan
|
|
}
|
|
tPrev := timestamps[0]
|
|
dSum := int64(0)
|
|
dMax := int64(dMaxs[rfa.idx] * 1000)
|
|
for _, t := range timestamps {
|
|
d := t - tPrev
|
|
if d <= dMax {
|
|
dSum += d
|
|
}
|
|
tPrev = t
|
|
}
|
|
return float64(dSum) / 1000
|
|
}
|
|
return rf, nil
|
|
}
|
|
|
|
func newRollupShareLE(args []interface{}) (rollupFunc, error) {
|
|
return newRollupShareFilter(args, countFilterLE)
|
|
}
|
|
|
|
func countFilterLE(values []float64, le float64) int {
|
|
n := 0
|
|
for _, v := range values {
|
|
if v <= le {
|
|
n++
|
|
}
|
|
}
|
|
return n
|
|
}
|
|
|
|
func newRollupShareGT(args []interface{}) (rollupFunc, error) {
|
|
return newRollupShareFilter(args, countFilterGT)
|
|
}
|
|
|
|
func countFilterGT(values []float64, gt float64) int {
|
|
n := 0
|
|
for _, v := range values {
|
|
if v > gt {
|
|
n++
|
|
}
|
|
}
|
|
return n
|
|
}
|
|
|
|
func countFilterEQ(values []float64, eq float64) int {
|
|
n := 0
|
|
for _, v := range values {
|
|
if v == eq {
|
|
n++
|
|
}
|
|
}
|
|
return n
|
|
}
|
|
|
|
func countFilterNE(values []float64, ne float64) int {
|
|
n := 0
|
|
for _, v := range values {
|
|
if v != ne {
|
|
n++
|
|
}
|
|
}
|
|
return n
|
|
}
|
|
|
|
func newRollupShareFilter(args []interface{}, countFilter func(values []float64, limit float64) int) (rollupFunc, error) {
|
|
rf, err := newRollupCountFilter(args, countFilter)
|
|
if err != nil {
|
|
return nil, err
|
|
}
|
|
return func(rfa *rollupFuncArg) float64 {
|
|
n := rf(rfa)
|
|
return n / float64(len(rfa.values))
|
|
}, nil
|
|
}
|
|
|
|
func newRollupCountLE(args []interface{}) (rollupFunc, error) {
|
|
return newRollupCountFilter(args, countFilterLE)
|
|
}
|
|
|
|
func newRollupCountGT(args []interface{}) (rollupFunc, error) {
|
|
return newRollupCountFilter(args, countFilterGT)
|
|
}
|
|
|
|
func newRollupCountEQ(args []interface{}) (rollupFunc, error) {
|
|
return newRollupCountFilter(args, countFilterEQ)
|
|
}
|
|
|
|
func newRollupCountNE(args []interface{}) (rollupFunc, error) {
|
|
return newRollupCountFilter(args, countFilterNE)
|
|
}
|
|
|
|
func newRollupCountFilter(args []interface{}, countFilter func(values []float64, limit float64) int) (rollupFunc, error) {
|
|
if err := expectRollupArgsNum(args, 2); err != nil {
|
|
return nil, err
|
|
}
|
|
limits, err := getScalar(args[1], 1)
|
|
if err != nil {
|
|
return nil, err
|
|
}
|
|
rf := func(rfa *rollupFuncArg) float64 {
|
|
// There is no need in handling NaNs here, since they must be cleaned up
|
|
// before calling rollup funcs.
|
|
values := rfa.values
|
|
if len(values) == 0 {
|
|
return nan
|
|
}
|
|
limit := limits[rfa.idx]
|
|
return float64(countFilter(values, limit))
|
|
}
|
|
return rf, nil
|
|
}
|
|
|
|
func newRollupHoeffdingBoundLower(args []interface{}) (rollupFunc, error) {
|
|
if err := expectRollupArgsNum(args, 2); err != nil {
|
|
return nil, err
|
|
}
|
|
phis, err := getScalar(args[0], 0)
|
|
if err != nil {
|
|
return nil, err
|
|
}
|
|
rf := func(rfa *rollupFuncArg) float64 {
|
|
bound, avg := rollupHoeffdingBoundInternal(rfa, phis)
|
|
return avg - bound
|
|
}
|
|
return rf, nil
|
|
}
|
|
|
|
func newRollupHoeffdingBoundUpper(args []interface{}) (rollupFunc, error) {
|
|
if err := expectRollupArgsNum(args, 2); err != nil {
|
|
return nil, err
|
|
}
|
|
phis, err := getScalar(args[0], 0)
|
|
if err != nil {
|
|
return nil, err
|
|
}
|
|
rf := func(rfa *rollupFuncArg) float64 {
|
|
bound, avg := rollupHoeffdingBoundInternal(rfa, phis)
|
|
return avg + bound
|
|
}
|
|
return rf, nil
|
|
}
|
|
|
|
func rollupHoeffdingBoundInternal(rfa *rollupFuncArg, phis []float64) (float64, float64) {
|
|
// There is no need in handling NaNs here, since they must be cleaned up
|
|
// before calling rollup funcs.
|
|
values := rfa.values
|
|
if len(values) == 0 {
|
|
return nan, nan
|
|
}
|
|
if len(values) == 1 {
|
|
return 0, values[0]
|
|
}
|
|
vMax := rollupMax(rfa)
|
|
vMin := rollupMin(rfa)
|
|
vAvg := rollupAvg(rfa)
|
|
vRange := vMax - vMin
|
|
if vRange <= 0 {
|
|
return 0, vAvg
|
|
}
|
|
phi := phis[rfa.idx]
|
|
if phi >= 1 {
|
|
return inf, vAvg
|
|
}
|
|
if phi <= 0 {
|
|
return 0, vAvg
|
|
}
|
|
// See https://en.wikipedia.org/wiki/Hoeffding%27s_inequality
|
|
// and https://www.youtube.com/watch?v=6UwcqiNsZ8U&feature=youtu.be&t=1237
|
|
bound := vRange * math.Sqrt(math.Log(1/(1-phi))/(2*float64(len(values))))
|
|
return bound, vAvg
|
|
}
|
|
|
|
func newRollupQuantiles(args []interface{}) (rollupFunc, error) {
|
|
if len(args) < 3 {
|
|
return nil, fmt.Errorf("unexpected number of args: %d; want at least 3 args", len(args))
|
|
}
|
|
tssPhi, ok := args[0].([]*timeseries)
|
|
if !ok {
|
|
return nil, fmt.Errorf("unexpected type for phi arg: %T; want string", args[0])
|
|
}
|
|
phiLabel, err := getString(tssPhi, 0)
|
|
if err != nil {
|
|
return nil, err
|
|
}
|
|
phiArgs := args[1 : len(args)-1]
|
|
phis := make([]float64, len(phiArgs))
|
|
phiStrs := make([]string, len(phiArgs))
|
|
for i, phiArg := range phiArgs {
|
|
phiValues, err := getScalar(phiArg, i+1)
|
|
if err != nil {
|
|
return nil, fmt.Errorf("cannot obtain phi from arg #%d: %w", i+1, err)
|
|
}
|
|
phis[i] = phiValues[0]
|
|
phiStrs[i] = fmt.Sprintf("%g", phiValues[0])
|
|
}
|
|
rf := func(rfa *rollupFuncArg) float64 {
|
|
// There is no need in handling NaNs here, since they must be cleaned up
|
|
// before calling rollup funcs.
|
|
values := rfa.values
|
|
if len(values) == 0 {
|
|
return nan
|
|
}
|
|
qs := getFloat64s()
|
|
qs.A = quantiles(qs.A[:0], phis, values)
|
|
idx := rfa.idx
|
|
tsm := rfa.tsm
|
|
for i, phiStr := range phiStrs {
|
|
ts := tsm.GetOrCreateTimeseries(phiLabel, phiStr)
|
|
ts.Values[idx] = qs.A[i]
|
|
}
|
|
putFloat64s(qs)
|
|
return nan
|
|
}
|
|
return rf, nil
|
|
}
|
|
|
|
func newRollupQuantile(args []interface{}) (rollupFunc, error) {
|
|
if err := expectRollupArgsNum(args, 2); err != nil {
|
|
return nil, err
|
|
}
|
|
phis, err := getScalar(args[0], 0)
|
|
if err != nil {
|
|
return nil, err
|
|
}
|
|
rf := func(rfa *rollupFuncArg) float64 {
|
|
// There is no need in handling NaNs here, since they must be cleaned up
|
|
// before calling rollup funcs.
|
|
values := rfa.values
|
|
phi := phis[rfa.idx]
|
|
qv := quantile(phi, values)
|
|
return qv
|
|
}
|
|
return rf, nil
|
|
}
|
|
|
|
func rollupHistogram(rfa *rollupFuncArg) float64 {
|
|
values := rfa.values
|
|
tsm := rfa.tsm
|
|
tsm.h.Reset()
|
|
for _, v := range values {
|
|
tsm.h.Update(v)
|
|
}
|
|
idx := rfa.idx
|
|
tsm.h.VisitNonZeroBuckets(func(vmrange string, count uint64) {
|
|
ts := tsm.GetOrCreateTimeseries("vmrange", vmrange)
|
|
ts.Values[idx] = float64(count)
|
|
})
|
|
return nan
|
|
}
|
|
|
|
func rollupAvg(rfa *rollupFuncArg) float64 {
|
|
// Do not use `Rapid calculation methods` at https://en.wikipedia.org/wiki/Standard_deviation,
|
|
// since it is slower and has no significant benefits in precision.
|
|
|
|
// There is no need in handling NaNs here, since they must be cleaned up
|
|
// before calling rollup funcs.
|
|
values := rfa.values
|
|
if len(values) == 0 {
|
|
// Do not take into account rfa.prevValue, since it may lead
|
|
// to inconsistent results comparing to Prometheus on broken time series
|
|
// with irregular data points.
|
|
return nan
|
|
}
|
|
var sum float64
|
|
for _, v := range values {
|
|
sum += v
|
|
}
|
|
return sum / float64(len(values))
|
|
}
|
|
|
|
func rollupMin(rfa *rollupFuncArg) float64 {
|
|
// There is no need in handling NaNs here, since they must be cleaned up
|
|
// before calling rollup funcs.
|
|
values := rfa.values
|
|
if len(values) == 0 {
|
|
// Do not take into account rfa.prevValue, since it may lead
|
|
// to inconsistent results comparing to Prometheus on broken time series
|
|
// with irregular data points.
|
|
return nan
|
|
}
|
|
minValue := values[0]
|
|
for _, v := range values {
|
|
if v < minValue {
|
|
minValue = v
|
|
}
|
|
}
|
|
return minValue
|
|
}
|
|
|
|
func rollupMax(rfa *rollupFuncArg) float64 {
|
|
// There is no need in handling NaNs here, since they must be cleaned up
|
|
// before calling rollup funcs.
|
|
values := rfa.values
|
|
if len(values) == 0 {
|
|
// Do not take into account rfa.prevValue, since it may lead
|
|
// to inconsistent results comparing to Prometheus on broken time series
|
|
// with irregular data points.
|
|
return nan
|
|
}
|
|
maxValue := values[0]
|
|
for _, v := range values {
|
|
if v > maxValue {
|
|
maxValue = v
|
|
}
|
|
}
|
|
return maxValue
|
|
}
|
|
|
|
func rollupTmin(rfa *rollupFuncArg) float64 {
|
|
// There is no need in handling NaNs here, since they must be cleaned up
|
|
// before calling rollup funcs.
|
|
values := rfa.values
|
|
timestamps := rfa.timestamps
|
|
if len(values) == 0 {
|
|
return nan
|
|
}
|
|
minValue := values[0]
|
|
minTimestamp := timestamps[0]
|
|
for i, v := range values {
|
|
// Get the last timestamp for the minimum value as most users expect.
|
|
if v <= minValue {
|
|
minValue = v
|
|
minTimestamp = timestamps[i]
|
|
}
|
|
}
|
|
return float64(minTimestamp) / 1e3
|
|
}
|
|
|
|
func rollupTmax(rfa *rollupFuncArg) float64 {
|
|
// There is no need in handling NaNs here, since they must be cleaned up
|
|
// before calling rollup funcs.
|
|
values := rfa.values
|
|
timestamps := rfa.timestamps
|
|
if len(values) == 0 {
|
|
return nan
|
|
}
|
|
maxValue := values[0]
|
|
maxTimestamp := timestamps[0]
|
|
for i, v := range values {
|
|
// Get the last timestamp for the maximum value as most users expect.
|
|
if v >= maxValue {
|
|
maxValue = v
|
|
maxTimestamp = timestamps[i]
|
|
}
|
|
}
|
|
return float64(maxTimestamp) / 1e3
|
|
}
|
|
|
|
func rollupTfirst(rfa *rollupFuncArg) float64 {
|
|
// There is no need in handling NaNs here, since they must be cleaned up
|
|
// before calling rollup funcs.
|
|
timestamps := rfa.timestamps
|
|
if len(timestamps) == 0 {
|
|
// Do not take into account rfa.prevTimestamp, since it may lead
|
|
// to inconsistent results comparing to Prometheus on broken time series
|
|
// with irregular data points.
|
|
return nan
|
|
}
|
|
return float64(timestamps[0]) / 1e3
|
|
}
|
|
|
|
func rollupTlast(rfa *rollupFuncArg) float64 {
|
|
// There is no need in handling NaNs here, since they must be cleaned up
|
|
// before calling rollup funcs.
|
|
timestamps := rfa.timestamps
|
|
if len(timestamps) == 0 {
|
|
// Do not take into account rfa.prevTimestamp, since it may lead
|
|
// to inconsistent results comparing to Prometheus on broken time series
|
|
// with irregular data points.
|
|
return nan
|
|
}
|
|
return float64(timestamps[len(timestamps)-1]) / 1e3
|
|
}
|
|
|
|
func rollupTlastChange(rfa *rollupFuncArg) float64 {
|
|
// There is no need in handling NaNs here, since they must be cleaned up
|
|
// before calling rollup funcs.
|
|
values := rfa.values
|
|
if len(values) == 0 {
|
|
return nan
|
|
}
|
|
timestamps := rfa.timestamps
|
|
lastValue := values[len(values)-1]
|
|
values = values[:len(values)-1]
|
|
for i := len(values) - 1; i >= 0; i-- {
|
|
if values[i] != lastValue {
|
|
return float64(timestamps[i+1]) / 1e3
|
|
}
|
|
}
|
|
if math.IsNaN(rfa.prevValue) || rfa.prevValue != lastValue {
|
|
return float64(timestamps[0]) / 1e3
|
|
}
|
|
return nan
|
|
}
|
|
|
|
func rollupSum(rfa *rollupFuncArg) float64 {
|
|
// There is no need in handling NaNs here, since they must be cleaned up
|
|
// before calling rollup funcs.
|
|
values := rfa.values
|
|
if len(values) == 0 {
|
|
// Do not take into account rfa.prevValue, since it may lead
|
|
// to inconsistent results comparing to Prometheus on broken time series
|
|
// with irregular data points.
|
|
return nan
|
|
}
|
|
var sum float64
|
|
for _, v := range values {
|
|
sum += v
|
|
}
|
|
return sum
|
|
}
|
|
|
|
func rollupRateOverSum(rfa *rollupFuncArg) float64 {
|
|
// There is no need in handling NaNs here, since they must be cleaned up
|
|
// before calling rollup funcs.
|
|
timestamps := rfa.timestamps
|
|
if len(timestamps) == 0 {
|
|
if math.IsNaN(rfa.prevValue) {
|
|
return nan
|
|
}
|
|
// Assume that the value didn't change since rfa.prevValue.
|
|
return 0
|
|
}
|
|
sum := float64(0)
|
|
for _, v := range rfa.values {
|
|
sum += v
|
|
}
|
|
return sum / (float64(rfa.window) / 1e3)
|
|
}
|
|
|
|
func rollupRange(rfa *rollupFuncArg) float64 {
|
|
max := rollupMax(rfa)
|
|
min := rollupMin(rfa)
|
|
return max - min
|
|
}
|
|
|
|
func rollupSum2(rfa *rollupFuncArg) float64 {
|
|
// There is no need in handling NaNs here, since they must be cleaned up
|
|
// before calling rollup funcs.
|
|
values := rfa.values
|
|
if len(values) == 0 {
|
|
return rfa.prevValue * rfa.prevValue
|
|
}
|
|
var sum2 float64
|
|
for _, v := range values {
|
|
sum2 += v * v
|
|
}
|
|
return sum2
|
|
}
|
|
|
|
func rollupGeomean(rfa *rollupFuncArg) float64 {
|
|
// There is no need in handling NaNs here, since they must be cleaned up
|
|
// before calling rollup funcs.
|
|
values := rfa.values
|
|
if len(values) == 0 {
|
|
return rfa.prevValue
|
|
}
|
|
p := 1.0
|
|
for _, v := range values {
|
|
p *= v
|
|
}
|
|
return math.Pow(p, 1/float64(len(values)))
|
|
}
|
|
|
|
func rollupAbsent(rfa *rollupFuncArg) float64 {
|
|
if len(rfa.values) == 0 {
|
|
return 1
|
|
}
|
|
return nan
|
|
}
|
|
|
|
func rollupPresent(rfa *rollupFuncArg) float64 {
|
|
// There is no need in handling NaNs here, since they must be cleaned up
|
|
// before calling rollup funcs.
|
|
if len(rfa.values) > 0 {
|
|
return 1
|
|
}
|
|
return nan
|
|
}
|
|
|
|
func rollupCount(rfa *rollupFuncArg) float64 {
|
|
// There is no need in handling NaNs here, since they must be cleaned up
|
|
// before calling rollup funcs.
|
|
values := rfa.values
|
|
if len(values) == 0 {
|
|
return nan
|
|
}
|
|
return float64(len(values))
|
|
}
|
|
|
|
func rollupStaleSamples(rfa *rollupFuncArg) float64 {
|
|
values := rfa.values
|
|
if len(values) == 0 {
|
|
return nan
|
|
}
|
|
n := 0
|
|
for _, v := range rfa.values {
|
|
if decimal.IsStaleNaN(v) {
|
|
n++
|
|
}
|
|
}
|
|
return float64(n)
|
|
}
|
|
|
|
func rollupStddev(rfa *rollupFuncArg) float64 {
|
|
return stddev(rfa.values)
|
|
}
|
|
|
|
func rollupStdvar(rfa *rollupFuncArg) float64 {
|
|
return stdvar(rfa.values)
|
|
}
|
|
|
|
func stddev(values []float64) float64 {
|
|
v := stdvar(values)
|
|
return math.Sqrt(v)
|
|
}
|
|
|
|
func stdvar(values []float64) float64 {
|
|
// See `Rapid calculation methods` at https://en.wikipedia.org/wiki/Standard_deviation
|
|
if len(values) == 0 {
|
|
return nan
|
|
}
|
|
if len(values) == 1 {
|
|
// Fast path.
|
|
return 0
|
|
}
|
|
var avg float64
|
|
var count float64
|
|
var q float64
|
|
for _, v := range values {
|
|
if math.IsNaN(v) {
|
|
continue
|
|
}
|
|
count++
|
|
avgNew := avg + (v-avg)/count
|
|
q += (v - avg) * (v - avgNew)
|
|
avg = avgNew
|
|
}
|
|
if count == 0 {
|
|
return nan
|
|
}
|
|
return q / count
|
|
}
|
|
|
|
func rollupIncreasePure(rfa *rollupFuncArg) float64 {
|
|
// There is no need in handling NaNs here, since they must be cleaned up
|
|
// before calling rollup funcs.
|
|
values := rfa.values
|
|
// restore to the real value because of potential staleness reset
|
|
prevValue := rfa.realPrevValue
|
|
if math.IsNaN(prevValue) {
|
|
if len(values) == 0 {
|
|
return nan
|
|
}
|
|
// Assume the counter starts from 0.
|
|
prevValue = 0
|
|
}
|
|
if len(values) == 0 {
|
|
// Assume the counter didn't change since prevValue.
|
|
return 0
|
|
}
|
|
return values[len(values)-1] - prevValue
|
|
}
|
|
|
|
func rollupDelta(rfa *rollupFuncArg) float64 {
|
|
// There is no need in handling NaNs here, since they must be cleaned up
|
|
// before calling rollup funcs.
|
|
values := rfa.values
|
|
prevValue := rfa.prevValue
|
|
if math.IsNaN(prevValue) {
|
|
if len(values) == 0 {
|
|
return nan
|
|
}
|
|
if !math.IsNaN(rfa.realPrevValue) {
|
|
// Assume that the value didn't change during the current gap.
|
|
// This should fix high delta() and increase() values at the end of gaps.
|
|
// See https://github.com/VictoriaMetrics/VictoriaMetrics/issues/894
|
|
return values[len(values)-1] - rfa.realPrevValue
|
|
}
|
|
// Assume that the previous non-existing value was 0
|
|
// only if the first value doesn't exceed too much the delta with the next value.
|
|
//
|
|
// This should prevent from improper increase() results for os-level counters
|
|
// such as cpu time or bytes sent over the network interface.
|
|
// These counters may start long ago before the first value appears in the db.
|
|
//
|
|
// This also should prevent from improper increase() results when a part of label values are changed
|
|
// without counter reset.
|
|
var d float64
|
|
if len(values) > 1 {
|
|
d = values[1] - values[0]
|
|
} else if !math.IsNaN(rfa.realNextValue) {
|
|
d = rfa.realNextValue - values[0]
|
|
}
|
|
if math.Abs(values[0]) < 10*(math.Abs(d)+1) {
|
|
prevValue = 0
|
|
} else {
|
|
prevValue = values[0]
|
|
values = values[1:]
|
|
}
|
|
}
|
|
if len(values) == 0 {
|
|
// Assume that the value didn't change on the given interval.
|
|
return 0
|
|
}
|
|
return values[len(values)-1] - prevValue
|
|
}
|
|
|
|
func rollupDeltaPrometheus(rfa *rollupFuncArg) float64 {
|
|
// There is no need in handling NaNs here, since they must be cleaned up
|
|
// before calling rollup funcs.
|
|
values := rfa.values
|
|
// Just return the difference between the last and the first sample like Prometheus does.
|
|
// See https://github.com/VictoriaMetrics/VictoriaMetrics/issues/1962
|
|
if len(values) < 2 {
|
|
return nan
|
|
}
|
|
return values[len(values)-1] - values[0]
|
|
}
|
|
|
|
func rollupIdelta(rfa *rollupFuncArg) float64 {
|
|
// There is no need in handling NaNs here, since they must be cleaned up
|
|
// before calling rollup funcs.
|
|
values := rfa.values
|
|
if len(values) == 0 {
|
|
if math.IsNaN(rfa.prevValue) {
|
|
return nan
|
|
}
|
|
// Assume that the value didn't change on the given interval.
|
|
return 0
|
|
}
|
|
lastValue := values[len(values)-1]
|
|
values = values[:len(values)-1]
|
|
if len(values) == 0 {
|
|
prevValue := rfa.prevValue
|
|
if math.IsNaN(prevValue) {
|
|
// Assume that the previous non-existing value was 0.
|
|
return lastValue
|
|
}
|
|
return lastValue - prevValue
|
|
}
|
|
return lastValue - values[len(values)-1]
|
|
}
|
|
|
|
func rollupDerivSlow(rfa *rollupFuncArg) float64 {
|
|
// Use linear regression like Prometheus does.
|
|
// See https://github.com/VictoriaMetrics/VictoriaMetrics/issues/73
|
|
_, k := linearRegression(rfa.values, rfa.timestamps, rfa.currTimestamp)
|
|
return k
|
|
}
|
|
|
|
func rollupDerivFast(rfa *rollupFuncArg) float64 {
|
|
// There is no need in handling NaNs here, since they must be cleaned up
|
|
// before calling rollup funcs.
|
|
values := rfa.values
|
|
timestamps := rfa.timestamps
|
|
prevValue := rfa.prevValue
|
|
prevTimestamp := rfa.prevTimestamp
|
|
if math.IsNaN(prevValue) {
|
|
if len(values) == 0 {
|
|
return nan
|
|
}
|
|
if len(values) == 1 {
|
|
// It is impossible to determine the duration during which the value changed
|
|
// from 0 to the current value.
|
|
// The following attempts didn't work well:
|
|
// - using scrape interval as the duration. It fails on Prometheus restarts when it
|
|
// skips scraping for the counter. This results in too high rate() value for the first point
|
|
// after Prometheus restarts.
|
|
// - using window or step as the duration. It results in too small rate() values for the first
|
|
// points of time series.
|
|
//
|
|
// So just return nan
|
|
return nan
|
|
}
|
|
prevValue = values[0]
|
|
prevTimestamp = timestamps[0]
|
|
} else if len(values) == 0 {
|
|
// Assume that the value didn't change on the given interval.
|
|
return 0
|
|
}
|
|
vEnd := values[len(values)-1]
|
|
tEnd := timestamps[len(timestamps)-1]
|
|
dv := vEnd - prevValue
|
|
dt := float64(tEnd-prevTimestamp) / 1e3
|
|
return dv / dt
|
|
}
|
|
|
|
func rollupIderiv(rfa *rollupFuncArg) float64 {
|
|
// There is no need in handling NaNs here, since they must be cleaned up
|
|
// before calling rollup funcs.
|
|
values := rfa.values
|
|
timestamps := rfa.timestamps
|
|
if len(values) < 2 {
|
|
if len(values) == 0 {
|
|
return nan
|
|
}
|
|
if math.IsNaN(rfa.prevValue) {
|
|
// It is impossible to determine the duration during which the value changed
|
|
// from 0 to the current value.
|
|
// The following attempts didn't work well:
|
|
// - using scrape interval as the duration. It fails on Prometheus restarts when it
|
|
// skips scraping for the counter. This results in too high rate() value for the first point
|
|
// after Prometheus restarts.
|
|
// - using window or step as the duration. It results in too small rate() values for the first
|
|
// points of time series.
|
|
//
|
|
// So just return nan
|
|
return nan
|
|
}
|
|
return (values[0] - rfa.prevValue) / (float64(timestamps[0]-rfa.prevTimestamp) / 1e3)
|
|
}
|
|
vEnd := values[len(values)-1]
|
|
tEnd := timestamps[len(timestamps)-1]
|
|
values = values[:len(values)-1]
|
|
timestamps = timestamps[:len(timestamps)-1]
|
|
// Skip data points with duplicate timestamps.
|
|
for len(timestamps) > 0 && timestamps[len(timestamps)-1] >= tEnd {
|
|
timestamps = timestamps[:len(timestamps)-1]
|
|
}
|
|
var tStart int64
|
|
var vStart float64
|
|
if len(timestamps) == 0 {
|
|
if math.IsNaN(rfa.prevValue) {
|
|
return 0
|
|
}
|
|
tStart = rfa.prevTimestamp
|
|
vStart = rfa.prevValue
|
|
} else {
|
|
tStart = timestamps[len(timestamps)-1]
|
|
vStart = values[len(timestamps)-1]
|
|
}
|
|
dv := vEnd - vStart
|
|
dt := tEnd - tStart
|
|
return dv / (float64(dt) / 1e3)
|
|
}
|
|
|
|
func rollupLifetime(rfa *rollupFuncArg) float64 {
|
|
// Calculate the duration between the first and the last data points.
|
|
timestamps := rfa.timestamps
|
|
if math.IsNaN(rfa.prevValue) {
|
|
if len(timestamps) < 2 {
|
|
return nan
|
|
}
|
|
return float64(timestamps[len(timestamps)-1]-timestamps[0]) / 1e3
|
|
}
|
|
if len(timestamps) == 0 {
|
|
return nan
|
|
}
|
|
return float64(timestamps[len(timestamps)-1]-rfa.prevTimestamp) / 1e3
|
|
}
|
|
|
|
func rollupLag(rfa *rollupFuncArg) float64 {
|
|
// Calculate the duration between the current timestamp and the last data point.
|
|
timestamps := rfa.timestamps
|
|
if len(timestamps) == 0 {
|
|
if math.IsNaN(rfa.prevValue) {
|
|
return nan
|
|
}
|
|
return float64(rfa.currTimestamp-rfa.prevTimestamp) / 1e3
|
|
}
|
|
return float64(rfa.currTimestamp-timestamps[len(timestamps)-1]) / 1e3
|
|
}
|
|
|
|
func rollupScrapeInterval(rfa *rollupFuncArg) float64 {
|
|
// Calculate the average interval between data points.
|
|
timestamps := rfa.timestamps
|
|
if math.IsNaN(rfa.prevValue) {
|
|
if len(timestamps) < 2 {
|
|
return nan
|
|
}
|
|
return (float64(timestamps[len(timestamps)-1]-timestamps[0]) / 1e3) / float64(len(timestamps)-1)
|
|
}
|
|
if len(timestamps) == 0 {
|
|
return nan
|
|
}
|
|
return (float64(timestamps[len(timestamps)-1]-rfa.prevTimestamp) / 1e3) / float64(len(timestamps))
|
|
}
|
|
|
|
func rollupChangesPrometheus(rfa *rollupFuncArg) float64 {
|
|
// There is no need in handling NaNs here, since they must be cleaned up
|
|
// before calling rollup funcs.
|
|
values := rfa.values
|
|
// Do not take into account rfa.prevValue like Prometheus does.
|
|
// See https://github.com/VictoriaMetrics/VictoriaMetrics/issues/1962
|
|
if len(values) < 1 {
|
|
return nan
|
|
}
|
|
prevValue := values[0]
|
|
n := 0
|
|
for _, v := range values[1:] {
|
|
if v != prevValue {
|
|
n++
|
|
prevValue = v
|
|
}
|
|
}
|
|
return float64(n)
|
|
}
|
|
|
|
func rollupChanges(rfa *rollupFuncArg) float64 {
|
|
// There is no need in handling NaNs here, since they must be cleaned up
|
|
// before calling rollup funcs.
|
|
values := rfa.values
|
|
prevValue := rfa.prevValue
|
|
n := 0
|
|
if math.IsNaN(prevValue) {
|
|
if len(values) == 0 {
|
|
return nan
|
|
}
|
|
prevValue = values[0]
|
|
values = values[1:]
|
|
n++
|
|
}
|
|
for _, v := range values {
|
|
if v != prevValue {
|
|
n++
|
|
prevValue = v
|
|
}
|
|
}
|
|
return float64(n)
|
|
}
|
|
|
|
func rollupIncreases(rfa *rollupFuncArg) float64 {
|
|
// There is no need in handling NaNs here, since they must be cleaned up
|
|
// before calling rollup funcs.
|
|
values := rfa.values
|
|
if len(values) == 0 {
|
|
if math.IsNaN(rfa.prevValue) {
|
|
return nan
|
|
}
|
|
return 0
|
|
}
|
|
prevValue := rfa.prevValue
|
|
if math.IsNaN(prevValue) {
|
|
prevValue = values[0]
|
|
values = values[1:]
|
|
}
|
|
if len(values) == 0 {
|
|
return 0
|
|
}
|
|
n := 0
|
|
for _, v := range values {
|
|
if v > prevValue {
|
|
n++
|
|
}
|
|
prevValue = v
|
|
}
|
|
return float64(n)
|
|
}
|
|
|
|
// `decreases_over_time` logic is the same as `resets` logic.
|
|
var rollupDecreases = rollupResets
|
|
|
|
func rollupResets(rfa *rollupFuncArg) float64 {
|
|
// There is no need in handling NaNs here, since they must be cleaned up
|
|
// before calling rollup funcs.
|
|
values := rfa.values
|
|
if len(values) == 0 {
|
|
if math.IsNaN(rfa.prevValue) {
|
|
return nan
|
|
}
|
|
return 0
|
|
}
|
|
prevValue := rfa.prevValue
|
|
if math.IsNaN(prevValue) {
|
|
prevValue = values[0]
|
|
values = values[1:]
|
|
}
|
|
if len(values) == 0 {
|
|
return 0
|
|
}
|
|
n := 0
|
|
for _, v := range values {
|
|
if v < prevValue {
|
|
n++
|
|
}
|
|
prevValue = v
|
|
}
|
|
return float64(n)
|
|
}
|
|
|
|
// getCandlestickValues returns a subset of rfa.values suitable for rollup_candlestick
|
|
//
|
|
// See https://github.com/VictoriaMetrics/VictoriaMetrics/issues/309 for details.
|
|
func getCandlestickValues(rfa *rollupFuncArg) []float64 {
|
|
currTimestamp := rfa.currTimestamp
|
|
timestamps := rfa.timestamps
|
|
for len(timestamps) > 0 && timestamps[len(timestamps)-1] >= currTimestamp {
|
|
timestamps = timestamps[:len(timestamps)-1]
|
|
}
|
|
if len(timestamps) == 0 {
|
|
return nil
|
|
}
|
|
return rfa.values[:len(timestamps)]
|
|
}
|
|
|
|
func getFirstValueForCandlestick(rfa *rollupFuncArg) float64 {
|
|
if rfa.prevTimestamp+rfa.window >= rfa.currTimestamp {
|
|
return rfa.prevValue
|
|
}
|
|
return nan
|
|
}
|
|
|
|
func rollupOpen(rfa *rollupFuncArg) float64 {
|
|
v := getFirstValueForCandlestick(rfa)
|
|
if !math.IsNaN(v) {
|
|
return v
|
|
}
|
|
values := getCandlestickValues(rfa)
|
|
if len(values) == 0 {
|
|
return nan
|
|
}
|
|
return values[0]
|
|
}
|
|
|
|
func rollupClose(rfa *rollupFuncArg) float64 {
|
|
values := getCandlestickValues(rfa)
|
|
if len(values) == 0 {
|
|
return getFirstValueForCandlestick(rfa)
|
|
}
|
|
return values[len(values)-1]
|
|
}
|
|
|
|
func rollupHigh(rfa *rollupFuncArg) float64 {
|
|
values := getCandlestickValues(rfa)
|
|
max := getFirstValueForCandlestick(rfa)
|
|
if math.IsNaN(max) {
|
|
if len(values) == 0 {
|
|
return nan
|
|
}
|
|
max = values[0]
|
|
values = values[1:]
|
|
}
|
|
for _, v := range values {
|
|
if v > max {
|
|
max = v
|
|
}
|
|
}
|
|
return max
|
|
}
|
|
|
|
func rollupLow(rfa *rollupFuncArg) float64 {
|
|
values := getCandlestickValues(rfa)
|
|
min := getFirstValueForCandlestick(rfa)
|
|
if math.IsNaN(min) {
|
|
if len(values) == 0 {
|
|
return nan
|
|
}
|
|
min = values[0]
|
|
values = values[1:]
|
|
}
|
|
for _, v := range values {
|
|
if v < min {
|
|
min = v
|
|
}
|
|
}
|
|
return min
|
|
}
|
|
|
|
func rollupModeOverTime(rfa *rollupFuncArg) float64 {
|
|
// There is no need in handling NaNs here, since they must be cleaned up
|
|
// before calling rollup funcs.
|
|
|
|
// Copy rfa.values to a.A, since modeNoNaNs modifies a.A contents.
|
|
a := getFloat64s()
|
|
a.A = append(a.A[:0], rfa.values...)
|
|
result := modeNoNaNs(rfa.prevValue, a.A)
|
|
putFloat64s(a)
|
|
return result
|
|
}
|
|
|
|
func getFloat64s() *float64s {
|
|
v := float64sPool.Get()
|
|
if v == nil {
|
|
v = &float64s{}
|
|
}
|
|
return v.(*float64s)
|
|
}
|
|
|
|
func putFloat64s(a *float64s) {
|
|
a.A = a.A[:0]
|
|
float64sPool.Put(a)
|
|
}
|
|
|
|
var float64sPool sync.Pool
|
|
|
|
type float64s struct {
|
|
A []float64
|
|
}
|
|
|
|
func rollupAscentOverTime(rfa *rollupFuncArg) float64 {
|
|
// There is no need in handling NaNs here, since they must be cleaned up
|
|
// before calling rollup funcs.
|
|
values := rfa.values
|
|
prevValue := rfa.prevValue
|
|
if math.IsNaN(prevValue) {
|
|
if len(values) == 0 {
|
|
return nan
|
|
}
|
|
prevValue = values[0]
|
|
values = values[1:]
|
|
}
|
|
s := float64(0)
|
|
for _, v := range values {
|
|
d := v - prevValue
|
|
if d > 0 {
|
|
s += d
|
|
}
|
|
prevValue = v
|
|
}
|
|
return s
|
|
}
|
|
|
|
func rollupDescentOverTime(rfa *rollupFuncArg) float64 {
|
|
// There is no need in handling NaNs here, since they must be cleaned up
|
|
// before calling rollup funcs.
|
|
values := rfa.values
|
|
prevValue := rfa.prevValue
|
|
if math.IsNaN(prevValue) {
|
|
if len(values) == 0 {
|
|
return nan
|
|
}
|
|
prevValue = values[0]
|
|
values = values[1:]
|
|
}
|
|
s := float64(0)
|
|
for _, v := range values {
|
|
d := prevValue - v
|
|
if d > 0 {
|
|
s += d
|
|
}
|
|
prevValue = v
|
|
}
|
|
return s
|
|
}
|
|
|
|
func rollupZScoreOverTime(rfa *rollupFuncArg) float64 {
|
|
// See https://about.gitlab.com/blog/2019/07/23/anomaly-detection-using-prometheus/#using-z-score-for-anomaly-detection
|
|
scrapeInterval := rollupScrapeInterval(rfa)
|
|
lag := rollupLag(rfa)
|
|
if math.IsNaN(scrapeInterval) || math.IsNaN(lag) || lag > scrapeInterval {
|
|
return nan
|
|
}
|
|
d := rollupLast(rfa) - rollupAvg(rfa)
|
|
if d == 0 {
|
|
return 0
|
|
}
|
|
return d / rollupStddev(rfa)
|
|
}
|
|
|
|
func rollupFirst(rfa *rollupFuncArg) float64 {
|
|
// There is no need in handling NaNs here, since they must be cleaned up
|
|
// before calling rollup funcs.
|
|
values := rfa.values
|
|
if len(values) == 0 {
|
|
// Do not take into account rfa.prevValue, since it may lead
|
|
// to inconsistent results comparing to Prometheus on broken time series
|
|
// with irregular data points.
|
|
return nan
|
|
}
|
|
return values[0]
|
|
}
|
|
|
|
func rollupDefault(rfa *rollupFuncArg) float64 {
|
|
values := rfa.values
|
|
if len(values) == 0 {
|
|
// Do not take into account rfa.prevValue, since it may lead
|
|
// to inconsistent results comparing to Prometheus on broken time series
|
|
// with irregular data points.
|
|
return nan
|
|
}
|
|
// Intentionally do not skip the possible last Prometheus staleness mark.
|
|
// See https://github.com/VictoriaMetrics/VictoriaMetrics/issues/1526 .
|
|
return values[len(values)-1]
|
|
}
|
|
|
|
func rollupLast(rfa *rollupFuncArg) float64 {
|
|
values := rfa.values
|
|
if len(values) == 0 {
|
|
// Do not take into account rfa.prevValue, since it may lead
|
|
// to inconsistent results comparing to Prometheus on broken time series
|
|
// with irregular data points.
|
|
return nan
|
|
}
|
|
return values[len(values)-1]
|
|
}
|
|
|
|
func rollupDistinct(rfa *rollupFuncArg) float64 {
|
|
// There is no need in handling NaNs here, since they must be cleaned up
|
|
// before calling rollup funcs.
|
|
values := rfa.values
|
|
if len(values) == 0 {
|
|
if math.IsNaN(rfa.prevValue) {
|
|
return nan
|
|
}
|
|
return 0
|
|
}
|
|
m := make(map[float64]struct{})
|
|
for _, v := range values {
|
|
m[v] = struct{}{}
|
|
}
|
|
return float64(len(m))
|
|
}
|
|
|
|
func rollupIntegrate(rfa *rollupFuncArg) float64 {
|
|
// There is no need in handling NaNs here, since they must be cleaned up
|
|
// before calling rollup funcs.
|
|
values := rfa.values
|
|
timestamps := rfa.timestamps
|
|
prevValue := rfa.prevValue
|
|
prevTimestamp := rfa.currTimestamp - rfa.window
|
|
if math.IsNaN(prevValue) {
|
|
if len(values) == 0 {
|
|
return nan
|
|
}
|
|
prevValue = values[0]
|
|
prevTimestamp = timestamps[0]
|
|
values = values[1:]
|
|
timestamps = timestamps[1:]
|
|
}
|
|
|
|
var sum float64
|
|
for i, v := range values {
|
|
timestamp := timestamps[i]
|
|
dt := float64(timestamp-prevTimestamp) / 1e3
|
|
sum += prevValue * dt
|
|
prevTimestamp = timestamp
|
|
prevValue = v
|
|
}
|
|
dt := float64(rfa.currTimestamp-prevTimestamp) / 1e3
|
|
sum += prevValue * dt
|
|
return sum
|
|
}
|
|
|
|
func rollupFake(rfa *rollupFuncArg) float64 {
|
|
logger.Panicf("BUG: rollupFake shouldn't be called")
|
|
return 0
|
|
}
|
|
|
|
func getScalar(arg interface{}, argNum int) ([]float64, error) {
|
|
ts, ok := arg.([]*timeseries)
|
|
if !ok {
|
|
return nil, fmt.Errorf(`unexpected type for arg #%d; got %T; want %T`, argNum+1, arg, ts)
|
|
}
|
|
if len(ts) != 1 {
|
|
return nil, fmt.Errorf(`arg #%d must contain a single timeseries; got %d timeseries`, argNum+1, len(ts))
|
|
}
|
|
return ts[0].Values, nil
|
|
}
|
|
|
|
func getIntNumber(arg interface{}, argNum int) (int, error) {
|
|
v, err := getScalar(arg, argNum)
|
|
if err != nil {
|
|
return 0, err
|
|
}
|
|
n := 0
|
|
if len(v) > 0 {
|
|
n = floatToIntBounded(v[0])
|
|
}
|
|
return n, nil
|
|
}
|
|
|
|
func getString(tss []*timeseries, argNum int) (string, error) {
|
|
if len(tss) != 1 {
|
|
return "", fmt.Errorf(`arg #%d must contain a single timeseries; got %d timeseries`, argNum+1, len(tss))
|
|
}
|
|
ts := tss[0]
|
|
for _, v := range ts.Values {
|
|
if !math.IsNaN(v) {
|
|
return "", fmt.Errorf(`arg #%d contains non-string timeseries`, argNum+1)
|
|
}
|
|
}
|
|
return string(ts.MetricName.MetricGroup), nil
|
|
}
|
|
|
|
func expectRollupArgsNum(args []interface{}, expectedNum int) error {
|
|
if len(args) == expectedNum {
|
|
return nil
|
|
}
|
|
return fmt.Errorf(`unexpected number of args; got %d; want %d`, len(args), expectedNum)
|
|
}
|
|
|
|
func getRollupFuncArg() *rollupFuncArg {
|
|
v := rfaPool.Get()
|
|
if v == nil {
|
|
return &rollupFuncArg{}
|
|
}
|
|
return v.(*rollupFuncArg)
|
|
}
|
|
|
|
func putRollupFuncArg(rfa *rollupFuncArg) {
|
|
rfa.reset()
|
|
rfaPool.Put(rfa)
|
|
}
|
|
|
|
var rfaPool sync.Pool
|